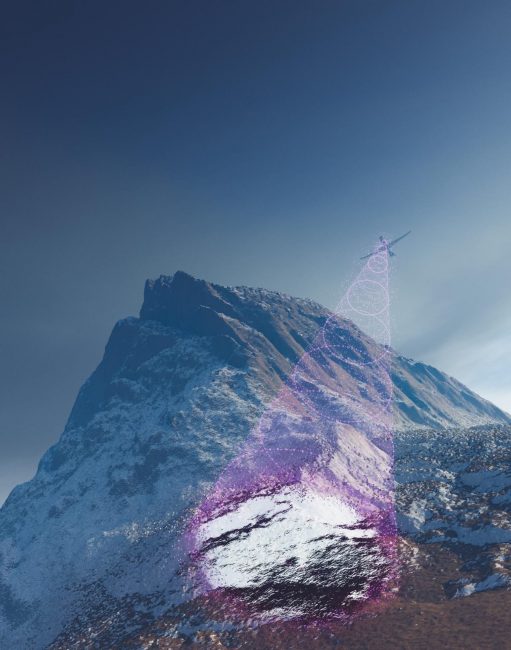
Artificial intelligence could help drones recognize and navigate terrain despite seasonal changes. Courtesy: California Institute of Technology.
Artificial intelligence could help drones recognize and navigate terrain regardless of how seasonal changes might alter its appearance, researchers say.
One way air and space robots can locate where they are without guidance from GPS or other external signals is a technique known as visual terrain-relative navigation. This strategy, first developed in the 1960s, compares what a robot sees of an area with previously collected high-resolution images. However, it doesn’t work as well when the area’s appearance changes due to seasonal variations in vegetation, lighting and snow cover.
Now scientists at the California Institute of Technology in Pasadena seek to improve this technique with a deep learning algorithm that removes superficial differences in a set of past and present images of a given area.
In the new study, the researchers trained AI software on visual datasets of the Rocky Mountains and parts of Connecticut. It learned to spot highly general abstract features of a region instead of landmarks tied to specific geographic locations. Consequently, it could navigate other areas with only small amounts of data.
“Computers can find obscure patterns that our eyes can’t see and can pick up even the smallest trend,” study co-author Connor Lee, a graduate student at Cal Tech, said in a statement.
The scientists tested their algorithm in a drone conducting a simulated flight over a region in northwest Connecticut. The area contained large uninterrupted expanses of rugged deciduous forest with steeper terrain than the places the algorithm encountered during training. Deciduous forests seasonally shed their leaves, and as such significantly change appearance over time. The steepness of the terrain is a challenge for the algorithm as well, as it can look significantly different depending on the altitude, angle and movements of the drone.
When the drone compared what it saw with photos taken two years beforehand, the algorithm eliminated nearly all substantial mismatches between the different sets of data. This helped the drone perform visual navigation successfully.
The researchers suggested their algorithm may also have applications for space missions. For example, the entry, descent and landing (EDL) system on JPL’s Mars 2020 Perseverance rover mission, used visual navigation to land at the Jezero Crater on the Red Planet, a site previously considered too hazardous for a safe entry. With rovers such as Perseverance, “a certain amount of autonomous driving is necessary, since transmissions could take 20 minutes to travel between Earth and Mars, and there is no GPS on Mars,” study senior author Soon-Jo Chung, a professor of aerospace and control and dynamical systems at Cal Tech, said in a statement.
The scientists will next see if their system can account for weather changes as well—fog, rain, snow and so on. If successful, their work could help improve navigation systems for driverless cars.
The researchers detailed their findings online June 23 in the journal Science Robotics.