To understand how dynamic neural networks function and why they matter for the future of autonomy, Inside Unmanned Systems spoke with Derek Whitley, CTO and co-founder of Vivum AI. Whitley discusses how Vivum’s approach differs from traditional AI models, where it is being deployed and how it supports robust autonomy in the real world.
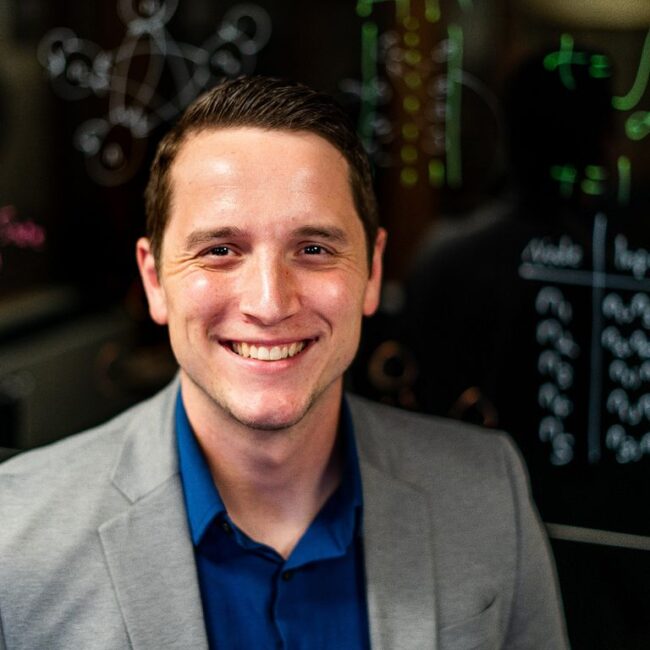
BIO: Derek Whitley is the CTO and co-founder of Vivum AI, a pioneering expert in Evolvable Hardware and Dynamic Neural Networks, Whitley applies evolutionary algorithms to reprogrammable hardware devices and leverages time series integration of artificial neural networks to generate complex and desirable behaviors. Prior to his corporate journey, he served in the United States Navy as a Cryptologic Technician. Academically, he holds a BS in Computer Programming from Strayer University, a Master’s in Informatics, and two Ph.Ds in Complex Systems and Cognitive Science from Indiana University Bloomington.
Artificial intelligence plays a vital role in unmanned systems, driving advancements in automation, perception and decision-making. While many AI-driven systems today rely on deep learning and structured automation, Vivum AI is taking a different path, leveraging dynamic neural networks to enable real-time adaptation and efficiency. This approach is designed to complement and enhance existing methodologies by introducing biologically inspired models that operate efficiently in constrained environments on UAVs and other autonomous platforms.
Whitley elucidates how these models work, their advantages over conventional AI approaches and their real-world applications in emergency recovery, last-mile delivery and BVLOS operations.
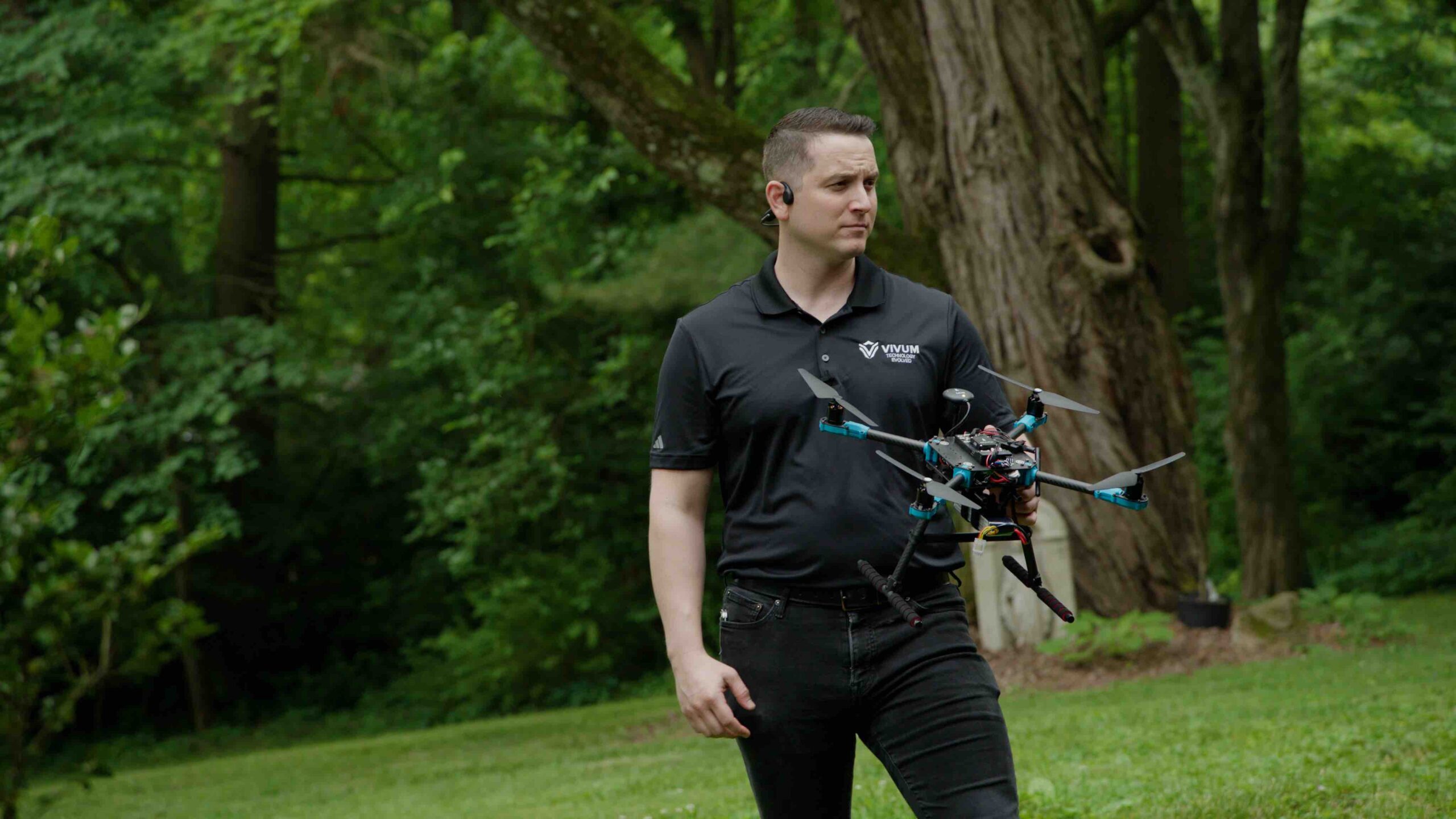
IUS: Let’s start with your background and what led you to co-found Vivum AI.
WHITLEY: I started my career in the United States Navy as a cryptologic technician. After leaving the service, I pursued a degree in computer science and software engineering and worked on embedded systems, developing electro-optic and infrared camera drivers, radar systems and low-power computing solutions. Eventually, I returned to academia and earned a dual PhD in complex systems and cognitive science from Indiana University Bloomington, where my research was funded by NASA.
That research focused on dynamic neural models—a type of artificial neural network that integrates sensory input over time, much like biological neurons. These models are highly efficient, adaptive and ideal for real-time decision-making in autonomous systems. At Vivum AI, we’ve built on that foundation, optimizing these models to run on low-power, constrained hardware—something that’s critical for unmanned systems operating in the field, underwater or in the air.
IUS: How does Vivum AI’s approach compare to deep learning?
WHITLEY: Traditional deep learning models are powerful but require large amounts of training data, significant computing power and dedicated GPUs or TPUs. They’re great for certain applications—like image classification or perception—but struggle when deployed in real-time systems with limited compute resources and power constraints.
Our dynamic neural networks, on the other hand, are designed to work in real-time with minimal computational overhead. Instead of processing information in discrete steps like deep learning, our models continuously integrate new data, allowing them to adapt dynamically to changing conditions.
Instead of pre-scripted automation, these neural models evolve behaviors organically, allowing systems to respond dynamically to changing conditions. It’s a fundamental rethinking of how AI should be structured for real-world autonomy.
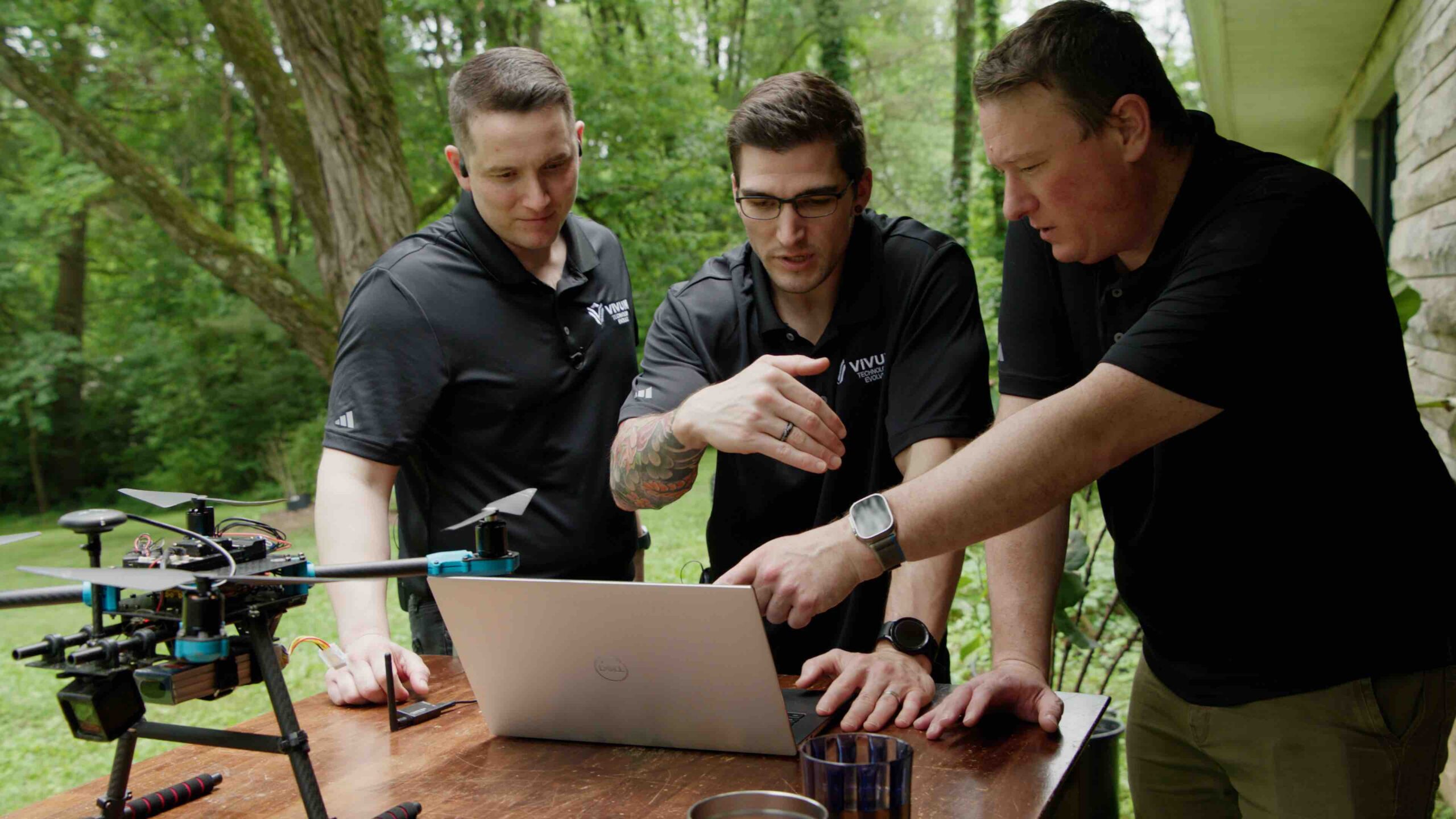
IUS: How do these models improve UAV reliability, particularly for beyond visual line of sight (BVLOS) operations?
WHITLEY: One of the biggest challenges in BVLOS operations is ensuring that a UAV can handle failures and respond to emergencies autonomously. Regulatory bodies like the FAA require safety redundancies, and one of the areas we’ve focused on is emergency response.
Why should a quadcopter that loses one motor automatically fail? There are four rotors. Losing one doesn’t mean the system has to catastrophically crash.
Most UAVs rely on PID controllers—predefined control loops that help maintain stability. These controllers work well under normal conditions, but when something unexpected happens—like a motor failure—they don’t always compensate effectively.
We’ve demonstrated a quadcopter that loses an entire motor and still lands safely. It doesn’t just fall out of the sky. It can even continue limited navigation using just three rotors. That’s a huge leap in reliability for BVLOS operations.
This type of adaptive control allows UAVs to recover from failures in real-time, making them safer and more resilient. It also helps with FAA waiver applications because regulators require UAVs to demonstrate fault tolerance before approving BVLOS operations.
IUS: How does this technology apply to commercial drone delivery?
WHITLEY: Last-mile delivery requires real-time navigation in unpredictable environments. Drones need to navigate through urban landscapes, avoid obstacles and deal with GPS limitations.
In urban environments, GPS can be unreliable and conditions change constantly. Traditional AI struggles in this environment because it’s trained for a specific, idealized scenario. Our models don’t just follow a fixed script. They react dynamically to what’s actually happening.
Vivum AI’s models allow drones to adjust their approach in real time, even when GPS signals are weak or obstacles appear unexpectedly. This is particularly valuable for delivery providers who need drones to safely approach doorsteps, avoid pedestrians and navigate tight spaces.
If a drone has to deliver a package to a front porch, it needs to figure out what to do if the planned drop site is obstructed. Our models allow for that level of adaptability without requiring expensive additional hardware.
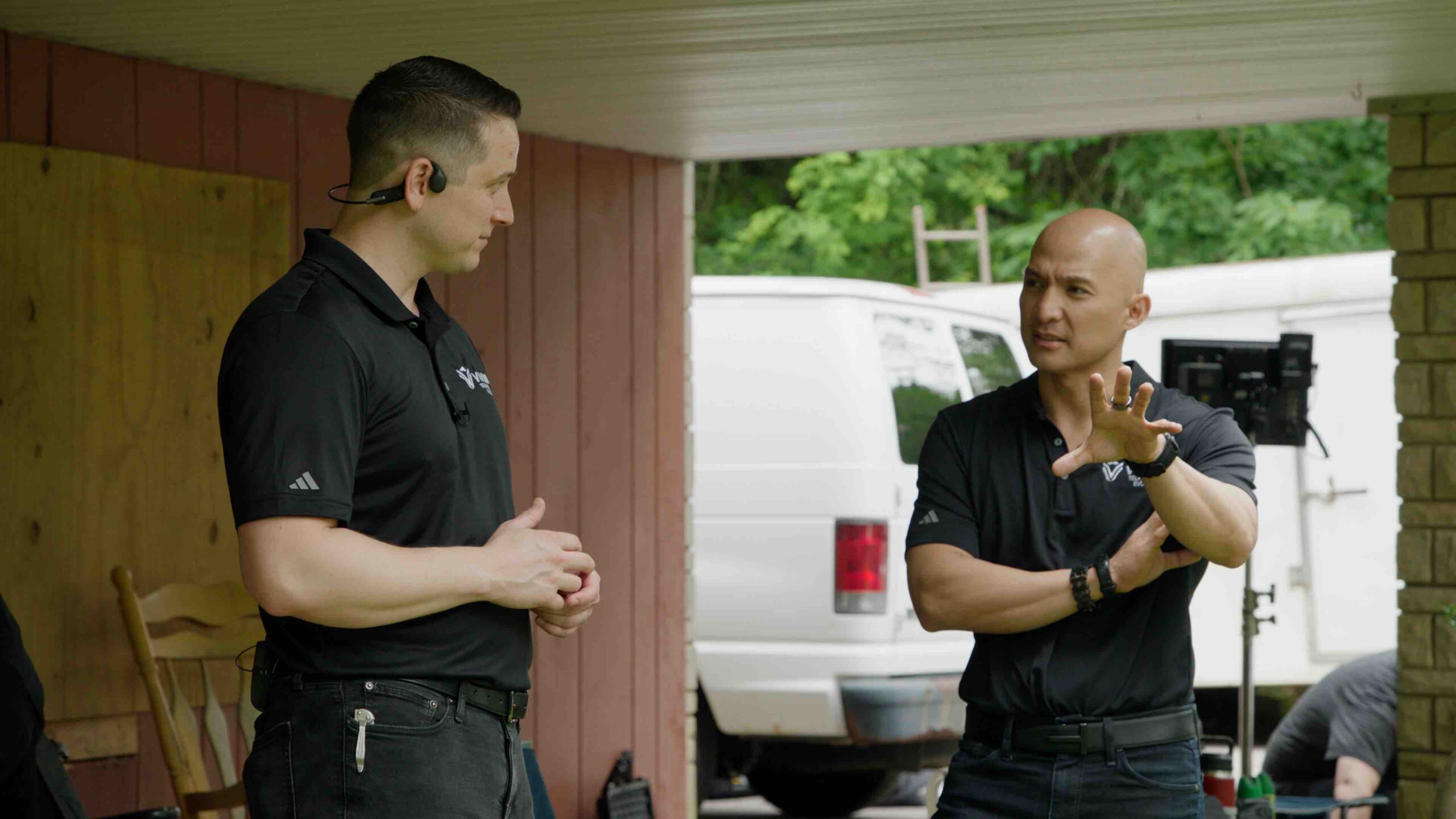
IUS: You’ve mentioned that Vivum AI focuses on behavior-driven autonomy rather than task-based. What does that mean?
WHITLEY: Most AI today is designed to execute specific tasks—for example, “fly from point A to point B while avoiding obstacles.” But what happens when the task needs to change on the fly?
A system of chained automation, or a series of if-then statements strung together with some lightweight control logic, is not true autonomy.
Instead of focusing solely on task execution, Vivum AI’s models develop adaptive behaviors that allow the system to generalize to new situations. This is particularly valuable in dynamic environments, where conditions can change rapidly, and rigid, pre-programmed responses may not suffice.
Our models exhibit emergent behaviors rather than rigidly following a pre-set list of instructions. That makes them far more adaptable to real-world conditions.
IUS: What’s the key takeaway for industry professionals considering Vivum AI’s approach?
WHITLEY: The biggest misconception in AI for unmanned systems is that better autonomy requires more hardware. While there are certainly cases where specialized compute resources add value, many of today’s systems could benefit from a different approach—smarter AI rather than bigger AI.
Instead of throwing more compute at the problem, we’re making AI more efficient. This is a fundamental shift in how AI is applied to real-world systems.
By optimizing AI models to run on existing flight controllers without requiring dedicated GPUs or high-power processors, Vivum AI’s technology enables more scalable, energy-efficient autonomy.
We’re at a turning point in unmanned systems. The future isn’t about making AI bigger. It’s about making it more efficient, more adaptive and more robust for real-world applications.
Whether for UAVs, ground robotics or underwater vehicles, the ability to operate in uncertain environments while conserving power is a game-changer.
As AI continues to evolve, a mix of approaches—including deep learning, structured automation and adaptive neural networks—will shape the future of unmanned systems. Vivum AI’s dynamic neural networks provide a new way forward, proving real-time adaptability, efficiency and resilience that can be achieved without massive computational overhead.
With applications ranging from BVLOS safety improvements to last-mile delivery and beyond, Vivum AI is pushing the boundaries of what autonomy truly means.