Founded with the aim of advancing how to use radar technology for security and intelligence-gathering use cases, MatrixSpace offers products that integrate real-time AI Edge processing, Radio Frequency (RF) communication and high-performance radar capabilities, in compact packages. Dr. Nihar Nanda leads AI product development for networked radar and AI-enabled sensors at MatrixSpace.
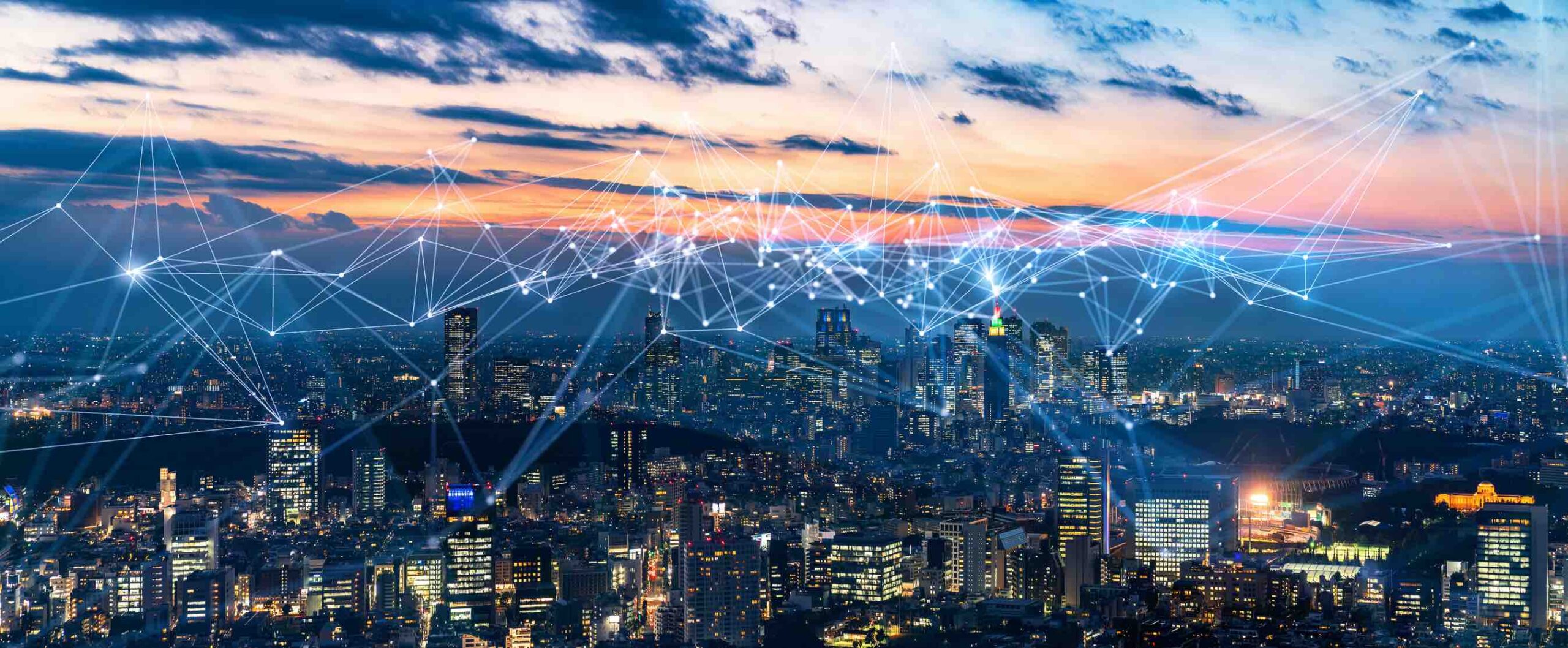
EDGE AI VS. ENTERPRISE AI
“My background is that I came from the AI and big data area. Most of my time was spent in developing AI algorithms and creating the big data product systems for enterprise customers,” Nanda told Inside Unmanned Systems. “I spent a lot of time doing enterprise AI, and from enterprise AI to AI at the edge is a very different challenge. I think solving edge AI problems for customers is actually more meaningful.”
Edge computing and edge AI refer to processing data locally on the actual device that registers it, at the “edge of the network,” instead of sending data to a cloud-based platform for processing. In the context of unmanned systems, that means having data processing and running AI models directly integrated with the sensors on a UAV. By cutting out the intermediary step of sending sensor data to the cloud for processing, systems can achieve more seamless communication to ground control or other receiving platforms. This increases the speed of data availability but faces software and hardware engineering challenges: the significantly reduced processing power that can be integrated directly into the drone, because of size and weight limitations, and the remaining latency between the sensor and the end user of the data.
Nanda said, “When you look at enterprise-level computing, the very first thing we think about is if there is enough compute available, and if there is enough capacity to run very large AI models.” Nanda continued, “Now, when we talk about edge computing there are two challenges. Number one is the current availability of compute. It’s very limited and we cannot put that enterprise compute power at the edge. So, I cannot run very large models. The second problem is latency. If we’re adding a sensor system, it sees something and it has to report very quickly about what it saw. What we at MatrixSpace are doing, is we are tackling those two elements in concert, and we are doing it completely outdoors.”
Nanda sees one of the advantages of edge computing as giving people the ability to perform higher-level tasks and more interesting work as a result of quicker sensor data availability. “Things like image recognition and LLM [large language models] and every other kind of huge compute, intensive AI technology is happening at the enterprise level. But there are so many things that we can do at the edge that can make human life much easier.”
“At MatrixSpace,” Nanda continued, “we wanted to put our technology into securing spaces. The radars are going to do the surveillance. Our first goal is to look after the [human] visual observers and the people who are on the rooftops looking out for things. It’s hard work to do. So, this is an example where I see that edge computing is the way to go. And our end goal is that we are truly committed to enabling edge computing, so that we can help people in doing their job” with greater safety and efficiency.
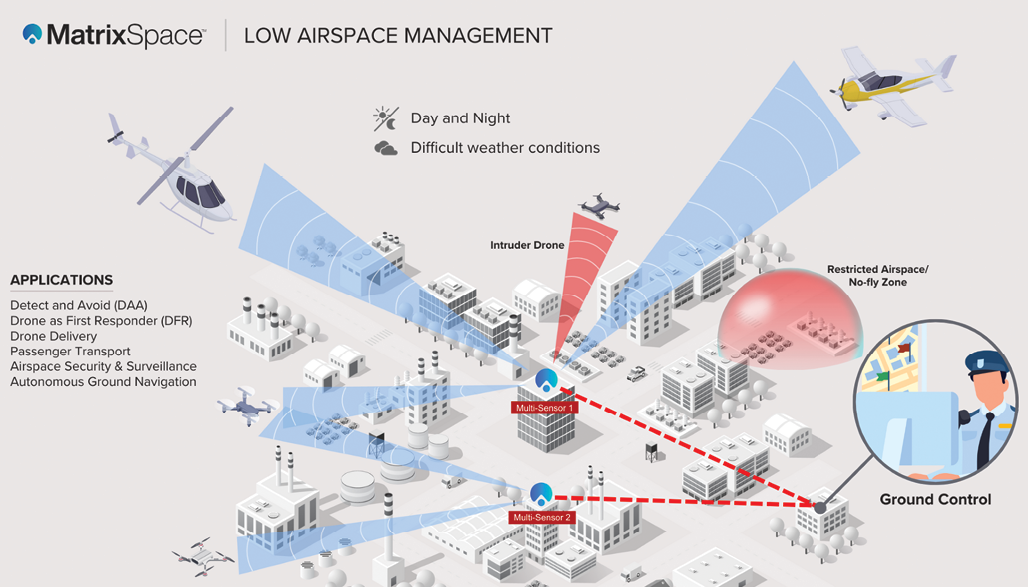
DATA MODEL TRAINING METHODOLOGY
Concerning how to approach these kinds of engineering problems, Nanda expanded on a methodology he calls, “right from the ground up.” MatrixSpace takes a unique approach to optimizing AI algorithms designed specifically to be used at the edge. “If you look at edge AI, developers use previously trained data models and downsize for edge applications. In some instances, this technique works but it often fails to perform because most of those models were never written to run with low compute and low latency. At MatrixSpace, we started with our own smaller models, added complexity, trained with real data and then optimized.”
Nanda explained the advantages of synthetic data for model training, followed by real data captured from outdoor sensors to improve prediction accuracy and reduce false positives. “There are many tools that can generate synthetic data from sophisticated environmental modeling. This is used to train the models initially, followed by model training with real-time data captured from sensors.”Nanda emphasized that “when the trained data encounters real-world sensing, predictions suffer in some cases. A model retraining with a small data set restores the performance. Just like the software gets updated, retrained model parameters get updated at the edge.”
“Our mission is to secure outdoor spaces,” Nanda explained. “Our approach to the problem stems from mimicking what a human observer would do, with a hugely accelerated capability to detect and track objects. When people secure outdoor spaces, the human agents stationed at strategic locations are vigilant, continuously observing surroundings for threats. As they listen for sounds and observe, they process in real time, creating hypotheses. And if they see something, they tell somebody. Once they do that, they may use binoculars to confirm further. This is the nature of human sensing for security. We try to model the technology to replace the human with intelligent sensors. So, when a radar scans a space, we need to find out what it sees, identify the object and estimate its position and movement. Our radar outputs 4D object data: the range, then the azimuth, elevation, and the velocity. Immediately after the scan, all observed objects are sent to a base station. When multiple sensors are observing the same scene, we use a sensor fusion technique to improve the observations of the surroundings. The base station assimilates the current observations with the historical observations to track object movements. Intelligent sensing at the edge in real-time continuously enables better decision making in threat assessment, evasive maneuvering and safety assessment.”
REAL-TIME OBJECT RECOGNITION AND TRACKING
“Images produce huge amounts of data. But what information is required to make safe navigation decisions is very little.” The AI-enabled radars at the edge, with object recognition and detection, narrow down and focus that decision making. “It works very efficiently while extending sensing capabilities to day and night conditions, smoke and fog,” Nanda explained. “Our edge radar AI takes gigabyte per second data generated from the radar and reduces that to kilobyte per second for decision-making.”
Concerning the frequently raised question of AI replacing human resources and jeopardizing current roles, Nanda offers the view that AI can enhance workforce creativity and improve workplace conditions. “So, don’t replace a person. The person who is sitting over there on the roof in a security firm, bring them inside, so that they can work comfortably.”
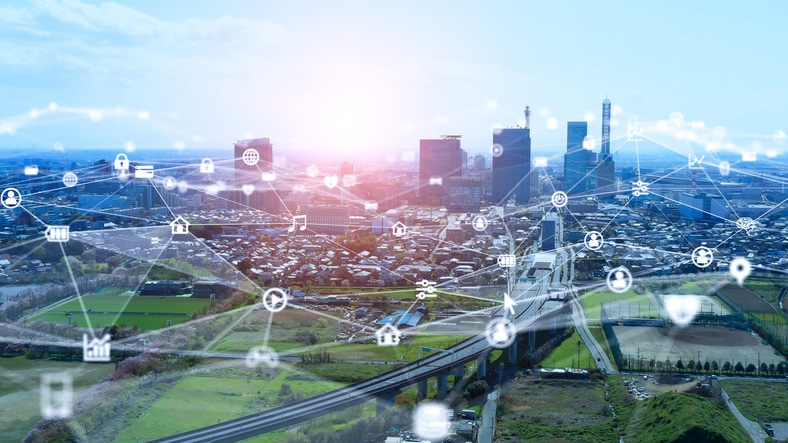
4D RADAR
Nanda provides further details on the MatrixSpace 4D radar’s capabilities, and what sets it apart. “Many radars provide just a range estimation, how far the object is from the radar. Along with range, it provides azimuth, angle from the center of the radar, elevation, how high the object is from the ground. Then it computes the velocity of that object. So, the radar data is richer. Knowing the current location of the object, trajectory AI models can predict where the object will be in the future. The advantages of 4D radar measurements are better estimation of current and future positions, better object identification from velocity and altitude.”
In terms of system integration, Nanda emphasized MatrixSpace radar hardware and software integrates easily with different platforms, whether mounted on drones or used in ground stations. “Our radar is designed to fit into multiple use cases, ground or moving platforms, the low SWAP-C and standard interfaces allow for quick integration. We believe in open API so that our customers can easily use radar as a component on their platforms, and integrate software. When the radar is mounted on an autonomous or manned system, the API provides the navigation system continuous situational awareness of the surroundings. The edge AI in the radar feeds real-time intelligence to the navigational systems to make informed decisions in a timely manner.”
INDUSTRY-LEVEL OBSERVATIONS
Speaking more broadly on the larger trends shaping the drone industry, Nanda emphasized we are currently in a pivotal moment for standardization in low airspace management. “This is the time when the industry is getting defined. The problem that we have is two-fold. One is we need a solution for low airspace management and secondly, we need a robust technology for low airspace surveillance. The FAA has the wisdom, and they can facilitate policy making, but the technology enablers are still under development. The policy part is tricky. Who actually owns and runs the low airspace surveillance—is it the city, the police department, or is it a pairing of agencies, or the military? Who wants that responsibility?”
Nanda highlighted the need for better sensing technology, reliable networking, and standardization in the unmanned systems industry. The evolution of these areas is crucial for the safe and efficient operation of AAM. “Unmanned systems are going to be the norm of the future. Nobody can deny that. But how we get there is in our hands, and we are defining it. The sensing technology is fundamental, with certainty the system needs to detect who is where, so that autonomous and semi-autonomous vehicles have situational awareness. For example, if I’m driving a car, I need to know where I am, where are other vehicles in the surrounding area, how fast are they moving and in what direction. Similarly, with unmanned systems, the autonomy engine needs the same information for safe navigation. Therefore, it is pivotal to have an active, real-time sensing system that covers vast areas and operates under every possible weather and atmospheric condition, without fail. The surveillance system of the future will use multi-modal sensor technologies with fusion providing ubiquitous views of the surroundings, which is only possible with modular design and open APIs.”
“Autonomous vehicles cannot operate without bi-directional communications, sharing their flight data with a central system and receiving situational awareness from the sensor system. It is very similar to ADSB but on steroids. Sensors and autonomous systems need networking, which will be a key enabler for this system,” Nanda continued. “All of these technology advancements need to happen at a rapid pace with tight collaboration. Today’s networking is still underdeveloped, we still have problems with cellular networks and so forth. Reliable network technology is a must-have for communications between unmanned systems.”
Along with networking and sensing in unmanned systems, questions arise on striking the optimal balance between data security and data sharing. “There has to be trust and security. Just because I can talk to you, it does not necessarily mean that I need to know who you are. But there does have to be a certain kind of identification or authentication that goes with that. So that we can have one unmanned system talk to another unmanned system, or one unmanned system talk to the ground control and then talk to another system. We don’t have all those mechanisms established yet. So that is from the technology perspective.”
Nanda believes unmanned systems will be a significant part of the future, but achieving this vision requires technological advancements, standardization, and collaborative efforts across the industry. Moving on to the perspective of “policy, the procedures, and the rules around” data security and sharing, Nanda asks, “What will be the protocol between these systems, how will they talk and communicate? So that is a big thing, an industry-wide revolution has to happen to enable the unmanned systems [networking]. And in this journey, there is not a single company that can say ‘we have everything.’ It’s much bigger than one government, much bigger than one single company.”
FUTURE ASPIRATIONS
Describing the business model and innovation philosophy of MatrixSpace, Nanda said, “our primary goal is to make sure we do innovate technology at a rapid rate, and we then find the gaps in the market. We are very carefully looking for what is changing in the market and what new regulations are on the horizon. And just like we built our first radar system within two years, which was [then] a gap, we are identifying a few technology areas where, within that area, we could use AI [to innovate today].
Detailing the types of innovations MatrixSpace looks to implement, “there’s simple AI which can do object identification and it goes a long way in improving how sensors work. We are also working on the networking areas to improve how there can be reliable communication that can happen in between the sensors, because when you have unmanned systems, they need ultra reliable communication. Otherwise, they cannot operate, they need to continuously be in chat with other systems.”