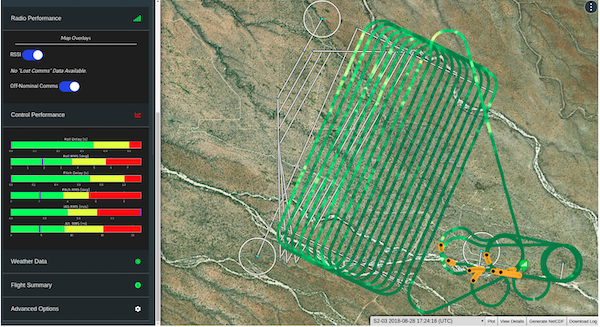
Screenshot of information delivered on the BST Dashboard.
Despite their low cost and high mission capability, dozens of RPA/UAS have crashed due to pilot error and mechanical and/or electrical failure. To improve on identifying potential failures of critical systems on small UAS, the USAF has awarded Black Swift Technologies (BST) an SBIR Grant to develop a machine learning software solution for predicting and improving UAS maintenance schedules.
“System failures can be costly—in time, money and equipment,” Black Swift Technologies CEO Jack Elston said in a release. “Our solution uses unsupervised learning for anomaly detection, which leverages algorithms that can build a model of how an aircraft should behave across a wide range of missions and flight conditions, then watch for instances that violate this models.”
The solution offered by the Boulder-based company relies on those algorithms to provide early warning and diagnostics of potential critical system failures on small UAS. Critical data is gathered from avionics data that the USAF already collects. If this data proves insufficient, BST has developed a set of monitoring nodes installed aboard candidate platforms to supplement the data sets and implement ML algorithms for real-time analysis and feedback.
Currently, many UAS users rely on limited-scope owner’s manuals. Even maintenance logs can lack subsystem state information. And critical components such as servos are often open-loop and unmonitored.
Black Swift Technologies’ solution sets out to fill those gaps without injecting undue complexity. “We use a web-based delivery platform consisting of a high-level and simplified red/yellow/green diagnostic rating for each subsystems,” Elson explained. “Each subsystem can be further explored, allowing the user to drill down into and retrieve more detailed information.” An easy-to-read dashboard provides decision-qualify information that is accessible to everyday end users.
The BST system tracks a growing number of known failures with labelled telemetry data. This supervised statistical learning can tie onboard telemetry to failures or maintenance concerns with, for example, servo motors and propellers, as well as to concerns produced by icing and other severe weather conditions. Proper grounding and maintenance can preclude systems failures. Unsupervised learning also is being employed to flag an aircraft for maintenance due to performance being “out of family.”
The outcome is improved awareness of the physical condition of a UAS and all its critical subsystems, which enhances reliability and mission-readiness.
Image courtesy of Black Swift Technologies.