As wind energy expands globally, maintaining the efficiency and longevity of turbine blades is a critical challenge. Traditional inspection methods—whether ground-based cameras, rope-access technicians, or LiDAR scans—often come with limitations in resolution, coverage, and operational efficiency.
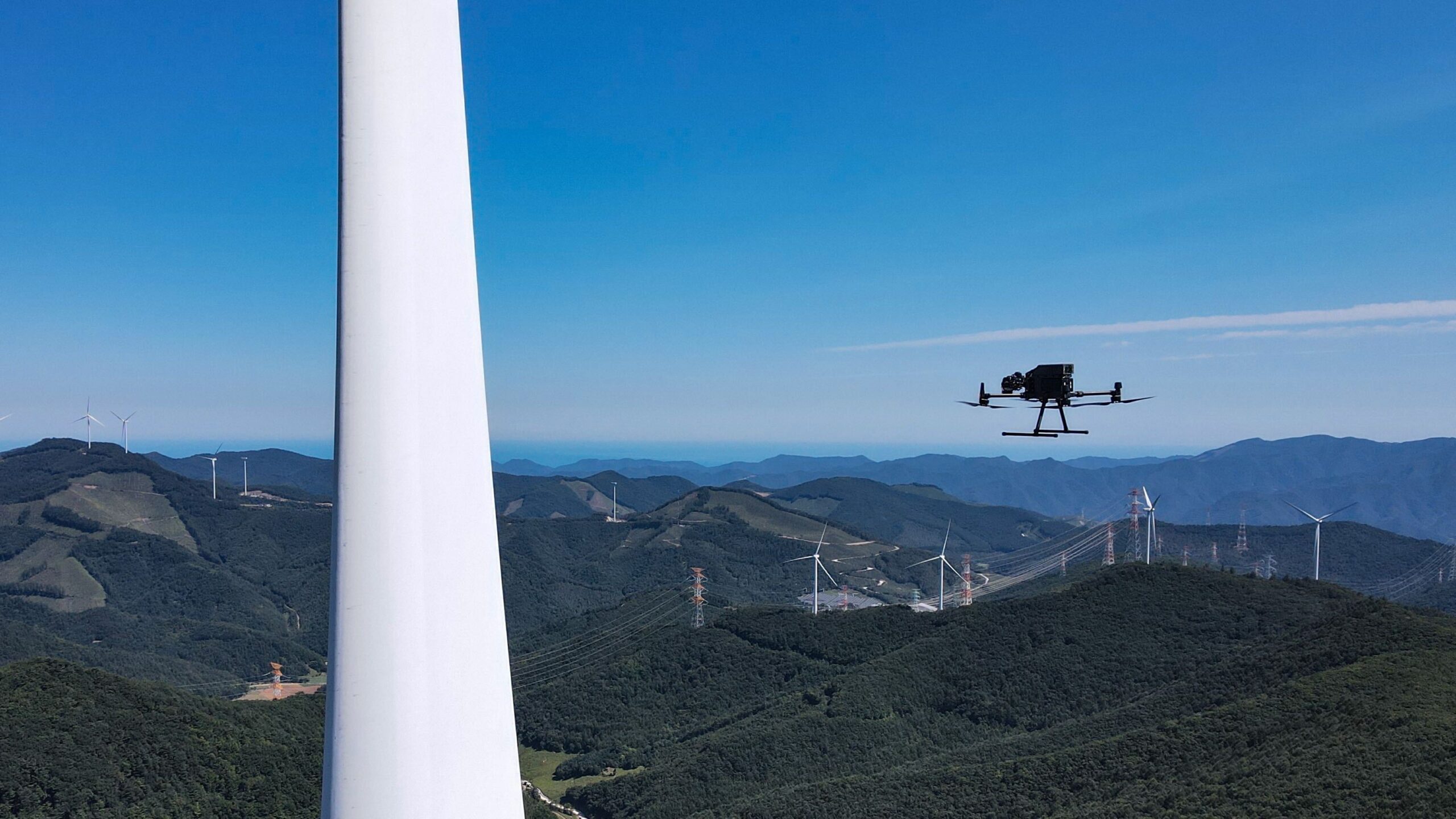
Nearthlab, a developer and provider of autonomous aerial inspection technology, is changing that equation.
Leveraging AI-driven drones, high-resolution imaging and proprietary data analytics through its Zoomable platform, Nearthlab is achieving faster, more precise and cost-effective wind turbine inspections.
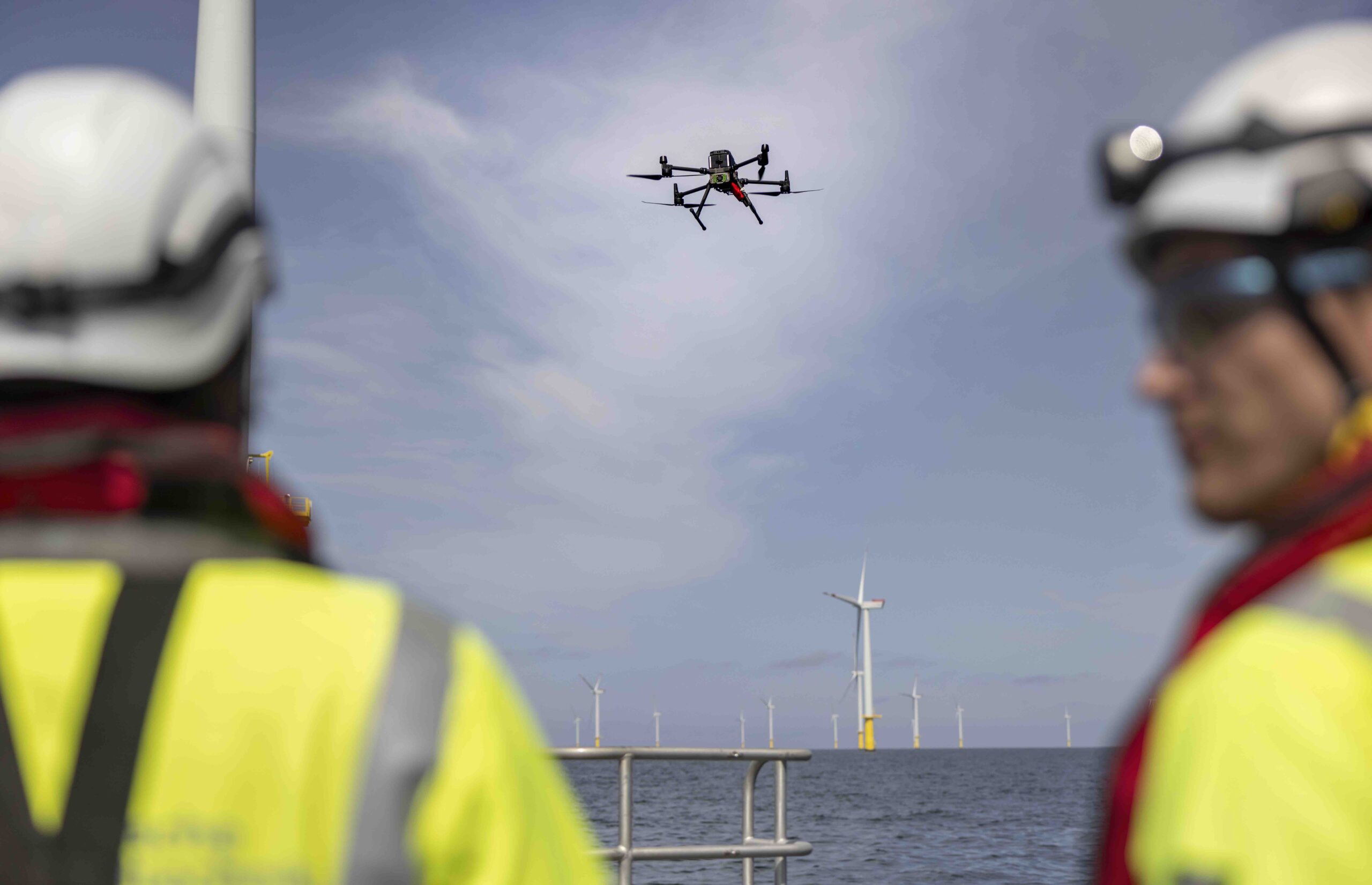
With deployments in more than 30 countries, the company’s autonomous solutions are helping wind farm operators optimize blade maintenance strategies, predict damage progression and minimize downtime, while reducing operational risk.
Inside Unmanned Systems connected with Hajun Kwon, head of wind business at the South Korea-based company for a deep dive into their proprietary software and airframe, AI solutions and future vision for wind farm inspections.
IUS: What are the primary sensor payloads used in your wind turbine inspection drones, and how do they compare in resolution and accuracy to traditional ground-based methods?
KWON: Nearthlab’s wind turbine inspection drones primarily use high-resolution cameras (64MP) with proprietary vision AI to capture detailed imagery of turbine blades. Compared to traditional ground-based methods (e.g., telescopic cameras or ground-based LiDAR), Nearthlab’s drones provide:
• Higher resolution: Close-range imaging ensures sub-millimeter accuracy, surpassing ground-based cameras that lose resolution at greater distances.
• Better angles: Drones capture blade surfaces from multiple perspectives, avoiding occlusions common in ground-based methods.
• Efficiency: Automated flight paths enable complete inspections in under 15 minutes, significantly reducing downtime.
IUS: Can you detail the AI-driven fault detection algorithms used in your inspection process?
KWON: Nearthlab’s AI-driven fault detection leverages deep learning models trained on extensive datasets of wind turbine blade defects. The AI:
• Identifies and classifies defects such as cracks, erosion and delamination.
• Uses anomaly detection algorithms to flag deviations from normal wear patterns.
• Continuously improves through machine learning as more inspection data is collected.
• With inspections conducted in over 30 countries across five continents, AI benefits from a diverse and expansive dataset, enabling it to adapt to various environmental and operational conditions.
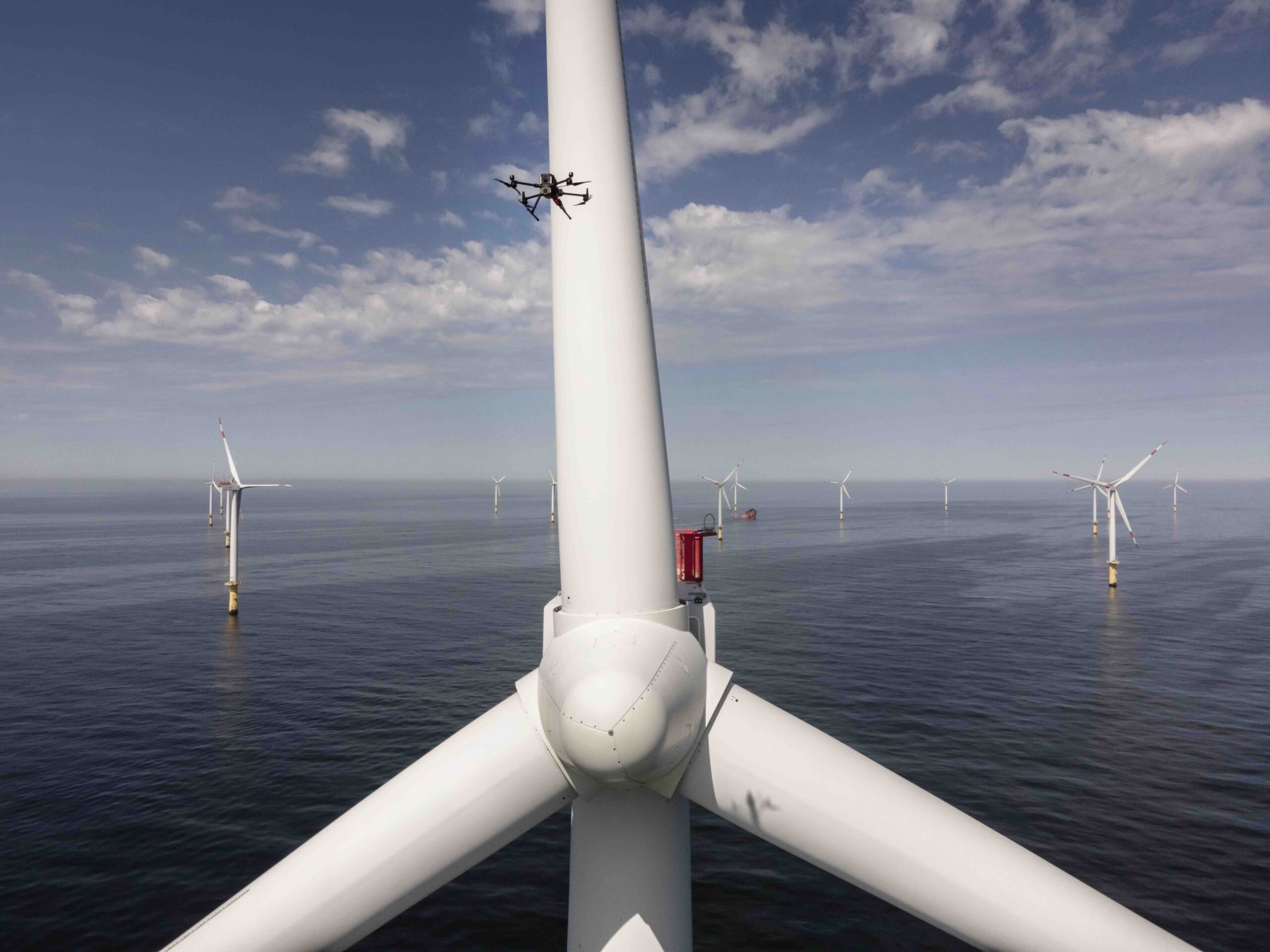
IUS: How does your system dynamically adjust flight paths for optimal coverage of turbine blades under variable wind conditions?
KWON: Our system dynamically adjusts flight paths for optimal coverage of turbine blades under variable wind conditions by leveraging high-precision LiDAR to accurately detect blade positions. We implement low-level control commands while directly developing high-level position control, ensuring precise maneuverability.
One of Nearthlab’s key strengths is having aerospace control engineers who specialize in this type of advanced flight control, allowing for seamless adaptation to changing wind conditions while maintaining optimal inspection coverage.
IUS: What defect classification taxonomy does Nearthlab use, and how does it ensure consistency in identifying delamination, leading-edge erosion and structural cracks?
KWON: Nearthlab utilizes a proprietary defect classification taxonomy developed based on extensive accumulated data, implemented within Zoomable, our drone data analytics platform. We have implemented a categorization system in Zoomable, where defects are classified from Severity Level 1 (minor contamination) to Severity Level 5 (immediate turbine shutdown).
These classifications are visually represented using intuitive colors, making it easy for even first-time users to quickly interpret and act upon the findings. By leveraging internally established standards, the platform ensures consistency in defect identification. It enables precise and reliable analysis across different inspection scenarios while enhancing efficiency and decision-making in blade maintenance and monitoring.
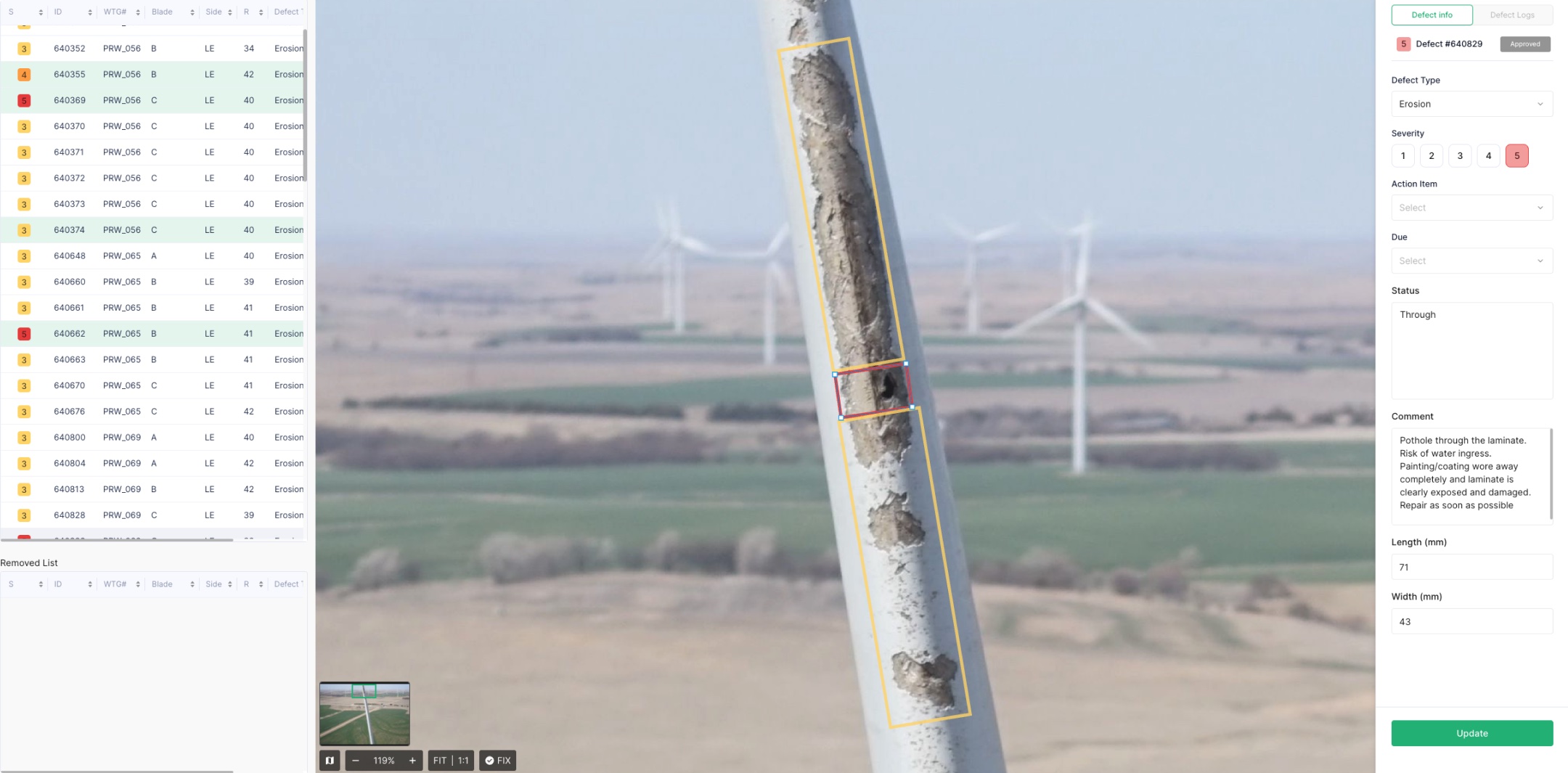
IUS: Does Nearthlab incorporate multi-sensor fusion (LiDAR, thermal, RGB, hyperspectral) to enhance defect detection, and how are these data streams integrated?
KWON: Nearthlab incorporates multi-sensor fusion to enhance defect detection. During flight, our system utilizes GPS, LiDAR and IMU sensors to estimate the drone’s position relative to the turbine blade, ensuring precise data collection for accurately detecting defect locations and sizes.
All collected data is then uploaded to Zoomable, where it is systematically recorded and managed. Through Zoomable Worker, these diverse data streams are integrated in a centralized environment, enabling comprehensive analysis and defect assessment.
IUS: How much of the image processing and defect detection occurs onboard the UAV versus post-flight in the cloud, and what are the latency trade-offs?
KWON: Nearthlab’s onboard processing is solely dedicated to autonomous flight, while defect detection occurs post-flight in the cloud. This approach is based on the nature of wind turbine inspections, where immediate, real-time defect detection is not a critical requirement compared to applications such as search and rescue, license plate recognition, or wildfire detection, where rapid response is essential.
By performing defect detection post-flight in the cloud, Nearthlab ensures higher processing accuracy and reliability rather than prioritizing speed. Cloud-based processing enables more powerful computing capabilities than onboard systems, allowing for advanced tasks beyond defect detection, such as redundancy elimination, numerical calculations, and comprehensive data analysis in a single workflow. This trade-off enhances overall efficiency and precision in wind turbine inspections.
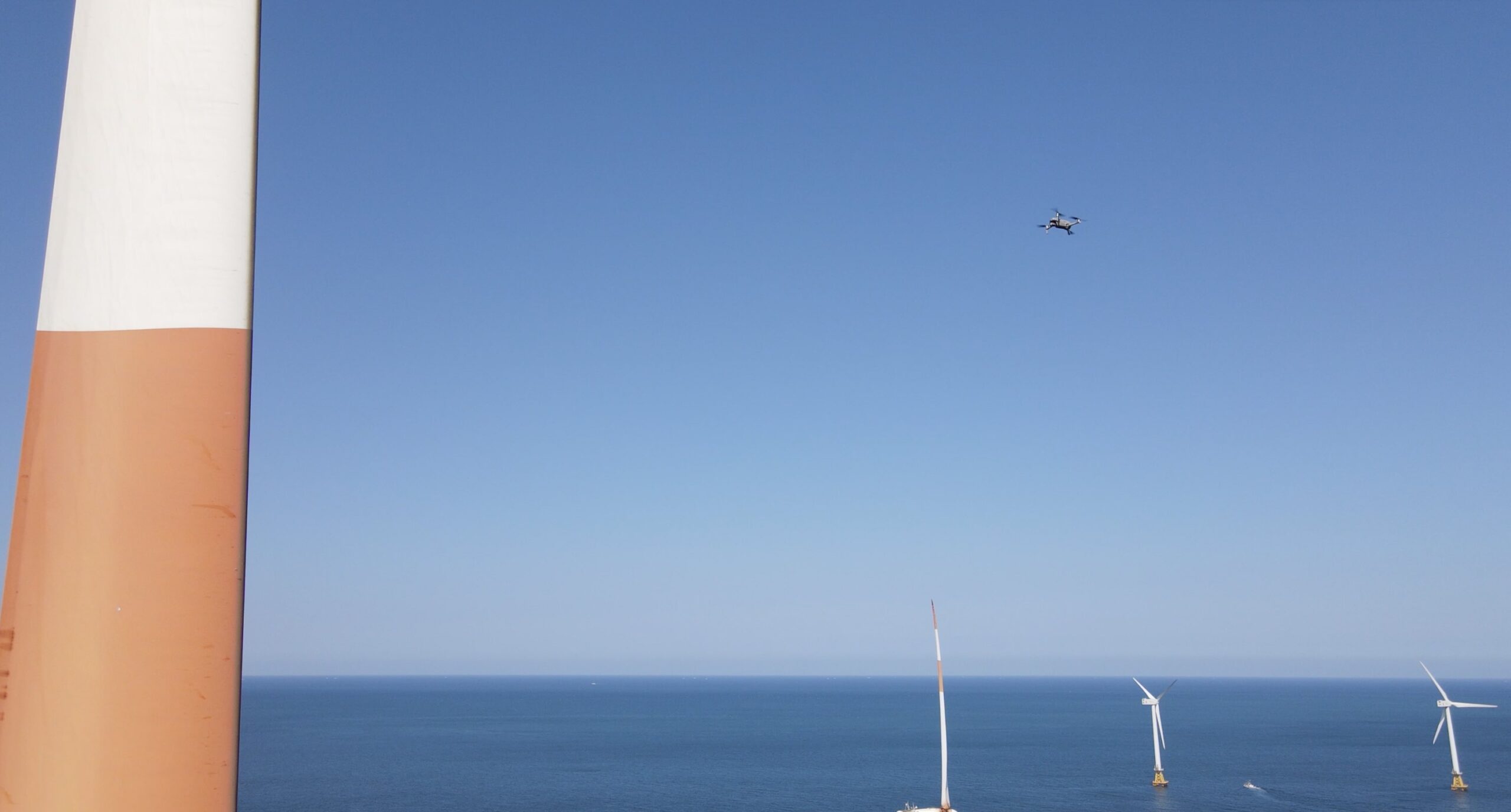
IUS: What GNSS-denied navigation capabilities does Nearthlab employ for offshore wind farm inspections where GPS signal degradation is common?
KWON: Our drones do not require GPS for flights, as they do not use waypoint missions. All navigation and flight control are conducted by an on-board vision-based processor that essentially enables the AI to look at the camera feed and determine the location of obstacles, just like a human eye would. This is the main flight technology used for GNC and there is a back-up LiDAR based system that is running in parallel so that the drone is able to continue its flight in case of one navigation system malfunction.
IUS: What is the maximum endurance of your UAVs in high-wind environments, and do you employ hybrid power sources or in-field battery-swapping solutions?
KWON: Our drones are built to withstand sustained wind speeds of up to 12 m/s and gusts of up to 14 m/s, ensuring reliable performance in challenging conditions. Because most onshore turbines have power sources at their base, we can efficiently charge our batteries on-site, reducing downtime between inspections.
For offshore operations, we utilize power sources inside the Crew Transfer Vessel (CTV) to recharge our devices. This allows us to conduct inspections seamlessly without interruptions caused by battery shortages.
IUS: Does Nearthlab support swarm-based drone inspections?
KWON: Not currently for drone inspections for wind turbines. However, leveraging our expertise in autonomous flight and AI-driven coordination, we have developed and are actively testing swarm-based drone technology for search and rescue missions and military applications—with AiDEN at the forefront. AiDEN is Nearthlab’s proprietary autonomous drone.
IUS: What communication protocols and bandwidth requirements does Nearthlab’s system use for transmitting high-resolution turbine inspection data in offshore scenarios?
KWON: Our incredibly strong track record in offshore turbine inspections stems from our powerful autonomous technology and strong pool of professional pilots. Close communication between our team and offshore technicians remains key in providing safe and efficient missions.
For the inspection data (images, videos), transmitting the data via wireless connections from the drone to the data management platform is ineffective due to limitations in the drone hardware and offshore internet speeds. As such, Nearthlab enforces a protocol where the images are stored on SSD and sent to the offshore wind farm site office or Nearthlab’s logistics center where a much faster internet upload is possible.
IUS: How does your inspection data feed into models for predictive maintenance of turbine blades?
KWON: Nearthlab is first able to provide an accurate assessment of the blade health by providing a comprehensive package of external, LPS and internal inspections. This ensures no damage goes undetected.
Within our data platform we are able to provide an additional feature of calculating the average energy production loss from external damage detected on the blade’s surface. This translates picture data into actionable financial information that can be used in establishing a short- and long-term operations and maintenance (O&M) strategy. Customers are able to accurately compare the cost of the repairs versus the additional revenue that can be harvested from having more aerodynamically efficient blades (through repairs).
Nearthlab was one of the first companies to provide a damage progression tracking feature on its data platform and has gathered a significant amount of damage progression data as a result. Using this data, we empirically predict when a particular damage will progress to the next severity level. Given that repair costs typically increase exponentially as the damage gets more severe, this feature allows users to make informed decisions about when to repair their blades.
IUS: Have you explored integrating vibration analysis or acoustic emission sensors to complement your visual inspections for component monitoring?
KWON: Within the renewables space, our speciality remains in visual inspections, software and autonomous technology. However, we have formed strong partnerships with global players who lead the industry in vibration analysis and acoustic emission sensors to offer a more complete package to customers who deem it necessary. This includes partnerships with CMS service providers.
Through the partnership, we are able to pool gearbox, drivetrain, pitch bearing, blades and all essential components of the wind turbine under a single umbrella to provide a truly comprehensive monitoring solution.
IUS: What role does environmental parameter monitoring (humidity, temperature, salt exposure) play in contextualizing defect severity in offshore wind inspections?
KWON: Damages progress differently depending on environmental conditions. For example, erosion damage tends to become a larger issue offshore due to salt exposure and the higher average wind level in offshore environments. These factors are already input into Nearthlab’s damage assessment AI and this ultimately translates into different recommended repair timelines. For example, a repair of a damage on an onshore blade may be recommended within 6 months while the identical damage on an offshore blade may be recommended within 3 months.
IUS: How does Nearthlab ensure compliance with BVLOS regulatory frameworks across different jurisdictions, and what onboard detect-and-avoid technologies are used?
NL: Nearthlab currently does not employ BVLOS operations for wind turbine inspections and the pilot periodically moves locations within the wind farm to maintain a constant line of sight. However, Nearthlab is currently reviewing the expansion of its offshore business to encompass BVLOS operations as the relevant regulations have become more welcoming to such operations.
IUS: What redundancies are built into your UAV systems to handle loss of GNSS or communication links in extreme weather conditions?
KWON: Nearthlab utilizes two navigation systems (vision, LiDAR) operating in parallel. This ensures there is always a fail-safe if one navigation system malfunctions.
The second mitigating factor is the enforcement of a strict HSE policy that dictates when pilots are able to operate the drones and when they are forbidden. This includes a detailed weather analysis when operating in less-than-ideal weather conditions to forecast if the weather conditions will further deteriorate during the mission.
More importantly, Nearthlab’s drones have a higher weather tolerance level compared to the vessels from which they operate with the exception of precipitation. If precipitation is present, all drone flights are grounded as rain drops on the camera lens would render the data unusable. Hence, the vessel captains typically make the decision to return to harbor before the weather deteriorates beyond drone-operable conditions.
IUS: How does Nearthlab integrate its datasets with SCADA systems and digital twin platforms used in wind energy asset management?
KWON: Nearthlab already provides a digital twin platform via its in-house data management platform Zoomable. Zoomable focuses on blades as a specialty, providing analytic tools for the exterior, interior and the LPS system of the blade.
When the customer wants an integration with SCADA systems, Nearthlab provides its Zoomable solution as a module that can be integrated with the customer’s existing SCADA system. This will require a bit of software integration with the SCADA provider, but Nearthlab has provided this integration to a number of SCADA providers.
IUS: What level of automation is built into the inspection-to-reporting workflow? Do you leverage AI to generate contextual maintenance recommendations?
KWON: When inspection data is uploaded to the Zoomable portal, Nearthlab’s AI will first review all images to ensure the captured images are in good condition. This includes checking for blur/out-of focus issues, lighting issues, full coverage of blades and missing metadata. The AI will also eliminate images that are redundant or unnecessary.
Afterwards, the AI will review all images and identify any anomalies that are identified on the blades. The AI will make an annotation box around the damage and categorize the damage pending on damage type and damage severity. The AI programming can be adjusted so the annotations are being made as per customized customer annotation guidelines. This ensures customers receive inspection reports as per their own standards, rather than that of the drone service provider.
IUS: How does Nearthlab contribute to condition-based maintenance (CBM) strategies, and what predictive analytics models are most effective?
KWON: Two major limitations in current O&M practices limit the implementation of a CBM O&M. The first is the lack of regular inspections. While many companies have adopted the practice of performing annual inspections, there are companies that will perform inspections every 2 or 4 years.
This limits a blade engineer’s ability to make informed decisions. There is also the issue of the time difference between the inspection and the repairs. Even if inspections are conducted annually, it often takes months before the actual repairs are executed based on the provided reports. This often leads to situations where damage hasdeteriorated significantly, and different repair methodologies are required.
Another issue is that severe damages are not properly monitored with the exception of low-quality binocular inspections from the ground. Employing a drone team to inspect one or two problematic turbines would cause O&M costs to skyrocket and that is why many customers cannot perform high-quality monitoring of severe damages that have not yet been repaired.
As a solution to both of these issues, Nearthlab launched its Mobile solution in 2022. This solution allows users to purchase recreational drones and perform autonomous inspections on their own using these drones. This is possible because Nearthlab has packaged its autonomous drone flight technology into an app that can be installed on drone controllers.
Using this solution, novice drone operators are able to perform spot inspections whenever required. This essentially means customers can perform annual inspections at a fraction of the cost by doing it themselves using cheap drones and they can continuously monitor severe damage without incurring additional costs. More frequent drone inspections lead to a more in-depth analysis of damage progression and ultimately an improvement in how to manage the said damages.