Near Earth Autonomy, based in Pittsburgh, has played a pioneering role adapting vertical lift aircraft—small and large alike—for autonomous operations. CEO and Co-Founder Sanjiv Singh, Ph.D., discussed what it means to be an autonomy integrator, his approach toward deterministic and non-deterministic forms of autonomy, the future of autonomous vertical-lift logistics in the Army and Marine Corps, and his work with the Air Force on autonomy certification and aircraft inspection.
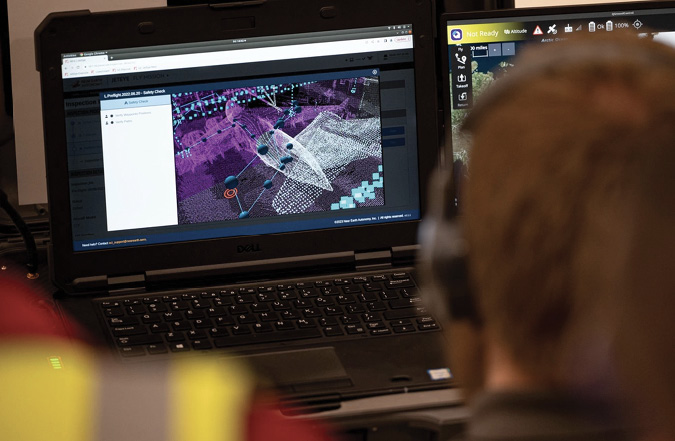
IUS: What would you list among Near Earth Autonomy’s most notable integration efforts?
SINGH: In 2010, we were on Boeing’s MD530F [light helicopter] research platform. It could take commands from a computer, there’s a documentary on that. It was the first time anybody had flown a helicopter autonomously. The program that really got us going was a Huey UH-1 [the classic Vietnam-era medium helicopter] we automated for cargo delivery in 2017 for AACUS [Autonomous Aerial Cargo Utility Systems].
After that we worked on a Kaman K-MAX helicopter optimized for hovering. We showed how you could do takeoff to landing carrying cargo with that aircraft. We are working on an autonomous Sikorsky H-60 Blackhawk that’s a proof of concept. We’re also working on an autonomous L3Harris FVR-90 for blood delivery and delivering medicines onto a ship deck.
IUS: Near Earth has been particularly involved in the parallel efforts by the Army and Marine Corps to develop a spectrum of uncrewed aerial logistics systems, including the KARGO drone. What can you tell us about those?
SINGH: When you talk about logistics trails of putting boots on the ground anywhere, there’s a large requirement for food, water, medicine, non-consumables. All those need to be sent, sometimes ahead of the main force, and sustained through resupply. It’s said that logisticians win wars because if you can’t keep a force supplied with water and fuel there’s not much they can do. The Army and Marine Corps (through NavAir) have converged over the last decade on requirement based on who you’re supplying and what the distances are, resulting in a small/medium/large classification of cargo UAVs.
Near Earth Autonomy is working on all six of those separate use cases, which are programs of record.
These reflect the services’ different needs. Sometimes it’s the ammo or equipment, like generators, that need carrying, or the range/endurance. For example, 150 pounds over 10 miles is happening. The Army’s H-VTOL program is intended to be a multi-role platform with casualty evacuation (CASEVAC) capability.
Small logistics UAS are likely to be electric vehicles. Medium-lift has to be a ground-up design. Two companies are in the Marine Corps competition; we’re working with Kaman Airspace’s KARGO vehicle, which is being pitched for both Army and Marine Corps medium-lift programs. [The rival design by Phenix Solutions and Leidos is called Sea Onyx.]
The large option is going to be some kind of retrofitted helicopter. The Marine Corps and Army have different ideas. From our perspective, we either take a brand-new aircraft just being put into service and add the smart system to fly safely and efficiently, or we take an existing helicopter that’s being retrofitted through our autonomy system. With some of these aircraft [being converted to autonomous flight] they only have a mechanical interface, you can’t talk to them digitally. What we do is install mechanisms so you can manipulate those controls through a computer.
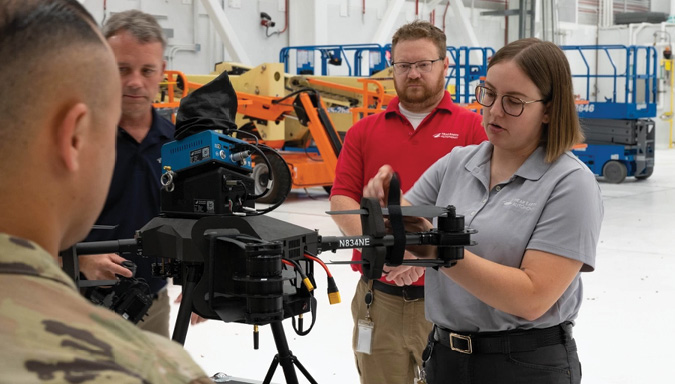
IUS: Might the Army’s fleet of retired OH-58 Kiowa helicopters appeal for conversion to unmanned logistics?
SINGH: Not the Kiowas, they don’t carry that much. What you want is either a Blackhawk or CH-47 Chinook, or CH-53 Sea Stallion. They’re in high demand. As the Blackhawks are gradually retired [replaced by forthcoming Bell V-280 tiltrotors], they will get upgraded as autonomous logistics aircraft. And they’ll inherit the Blackhawk’s robust spare parts supply chain.
IUS: What about Near Earth Autonomy’s work with the Air Force?
SINGH: The Air Force doesn’t need to do this kind of resupply operationally and is mostly interested in fixed-wing aircraft for autonomy. But the service’s AFWERX program is interested in realizing next generation autonomous aircraft that carry people or cargo anywhere from a few hundred pounds to a few thousand. AFWERX funds this development and accelerates the maturation of these new-generation aircraft.

While less interested in retrofitting autonomy, the Air Force does have a program called Agility Prime to operationally deploy by 2025 an electrically powered VTOL aircraft. There’s also Autonomy Prime aimed to help autonomous technologies transition into programs of record.
We are working with the Air Force to build a certification or accreditation framework that could apply to multiple aircraft. We develop aircraft agnostic autonomy. Of course, individual aircraft dynamics matter, but we’re able to adapt that to a wide range of aircraft, from hybrid VTOLs to fixed wing. For example, FVR90 by L3Harris has fixed rotors to go up and down and a push prop in the back [see Inside Unmanned Systems April/May 2024 issue for a feature on the FVR90].
We’ve put our autonomy on a wide range of aircraft that’s meant to plan routes for the aircraft most efficiently both while on the ground and in the air. Some of it is to watch for off-nominal conditions; there may be another aircraft in the vicinity and changing the flight path so as not to collide with it, or finding an alternate path if the place you’re supposed to land isn’t clear for some reason. The aircraft has to be intelligent.
We want to answer: ‘How reliable is it? Will the autonomy be there when you need it even when components fail?’ Of course, any subcomponent could fail, you still want it to be able to fail safely and still do the mission or be able to recover and return to base or in worst case ditch safely. That’s what autonomy is supposed to do, and it’s the scope of our work with the Air Force.
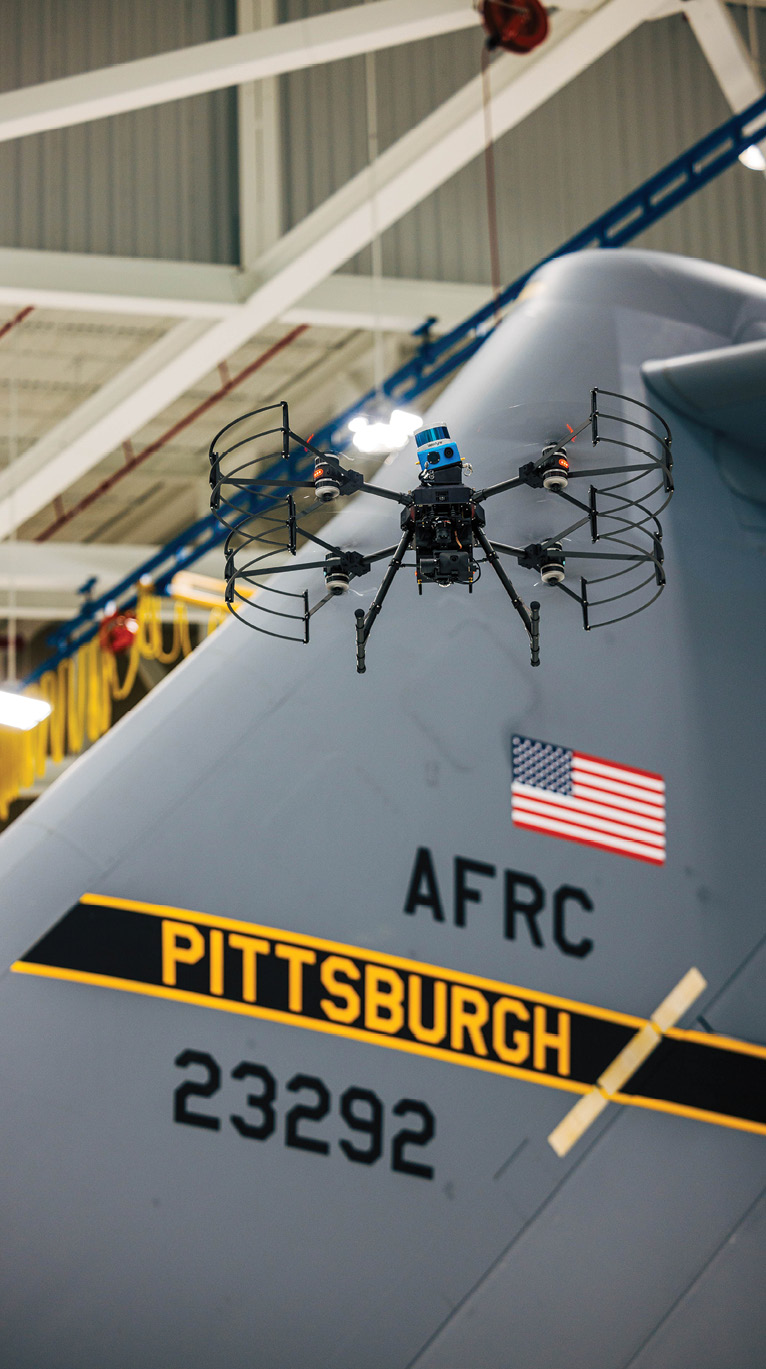
IUS: What methods do you take for measuring reliability—and achieving it?
SINGH: If you develop a system, that has been certified under the D0178 hardware and D0154 software standard—and it takes a long time to do so, as with autonomy, there are a lot of things that aren’t easy to do under those fixed standards. Especially for non-deterministic behavior.
You’re not going to be able to describe things to the T with autonomy. But there are techniques you can process (statistically, anecdotally) to make some estimate of failure rates. When you employ a system of systems, the bigger system has some idea of potential failures and expected success rates of the subordinate autonomous systems.
GPS already has a failure rate of 1 in 10,000 hours from various causes. We have to build up a case that this is the kind of reliability you can reasonably expect from autonomous platforms too, and how to mitigate these kinds of errors through solid systems engineering, so when we improve these systems, we don’t have to go all the way [back] to the start. Typically, you have to do whole re-certification whether it’s a one-line change to code or 10 lines. We want a new standard development process that’s easy to develop under, certify, adapt and improve over time. With this [development] technology, an original equipment manufacturer [OEM] can know the reliability rating of their autonomous platform.
IUS: As an autonomy integrator, how does the business model work when cooperating with platform builders? Are the fees one-time service/licensures for integrating autonomy onto a platform, or is there also per-unit-sold compensation?
SINGH: There’s a couple of models, depending on how much work is involved. We build autonomous model kits using a system called Paragon that’s very easy to attach to an aircraft and have it do some things autonomously. For people who know what they’re doing, we sell them the hardware/software.
Ideally, this progresses to a deeper level requiring more optimization, integration of technology including into the aircraft’s mission management architecture. How are you going to request it to do something? Or dispatch the platform, monitor it, and deal with contingencies? These are all the things that are necessary for eventual scaled usage. The model in that case is licensing; we sell them the hardware and help them make it work.

There are other models, including per airframe built or per hour of operation of the UAV. But generally, the bigger the aircraft, the more expensive the deal, the simpler you want the financial arrangement to be.
On the slower, low-cost side where creating value becomes really important, what you might want to do is help the company and provide the hardware for free, but then receive compensation for every safe landing. That way every time the platform lands, it’s monetized. Those are creative ways to help pay for the economics of autonomy recurringly.
IUS: Are you interested in neural networks and reinforcement learning AIs?
SINGH: We stay away from that kind of stuff. We’re very familiar with those techniques. They’re an industry buzzword. But the idea that every autonomous system must have AI, we don’t believe that at all. Most of our stuff doesn’t.
Yes, there are places where AI’s good. We’ve thought about it. Learning is useful for a system that needs to improve as there’s no other way to get it to autonomy without teaching it. Say, if you wanted to look for cancer in an MRI, you could go from first principles and have a system look for certain things, but there’s no descriptive kind of system that might work. You show a system hundreds of thousands of cases when cancer was detected, and the system learns from those examples.
In our world, that might mean I have an aircraft flying at low altitude, and there are several other aircraft in the vicinity. It’s very hard to detect those aircraft, but if you have cameras all around you, you might be able to pick up a little blip because I’ve seen hundreds of thousands of pictures of aircraft like that.
Recognition tasks are an example of what AI learning does well. Another use is when an aircraft is coming to land, you might use sensors to say “this is a person, or a vehicle” that’s too close to where I’m going to land. It’s not just any object, it’s an object of a particular type.
We generally stay away from these methods because they’re really hard to predict reliability rates for, but sometimes there’s no other method that will work.
IUS: So, you lean against the self-learning model because you’d prefer more predictable behaviors?
SINGH: Our major technique for perceiving the environment is just based on geometry and statistics. We separate the world into these little boxes, or voxels (3d pixels). We then shoot a laser 500,000 times a second—and wherever the laser ‘hits’ some little piece of the environment, that means something is there. When it doesn’t, there’s evidence that nothing is there. It’s not strictly binary, we use probability/statistics.
AI recognition is also used, but they’re a small part of our concept of operations. That’s in contrast to self-driving cars which need to figure out ‘what’s that I’m looking at, a pedestrian, bicyclist, mailbox, person bent over, on crutches, wheelchair, child etc.?’ Why? Because you need to predict where those objects are going to be the next few seconds. Some of these things are static, they’re not moving, which is useful to know. And if somebody’s standing, which way are they facing? That affects the likelihood of which direction they might move. Our own brains do this all the time when we’re driving.
But from an air vehicle perspective, we have to do fewer of those kinds of recognition problems—mostly pertaining to other moving aircraft.
IUS: NEA also has an aircraft inspection business. Or rather, autonomous aircraft inspecting other aircraft.
SINGH: That’s a totally different model. [For aircraft inspection] We’re a complete vertical, provide the whole solution for drones to inspect larger aircraft—military and commercial. That’s a very tightly regulated game, and we’re trying to make some impact in that area.
There’s a lot of interest in doing this for unscheduled maintenance. Say there’s a surge of demand after a hailstorm and there are 50 aircraft grounded until inspected—how quickly can you inspect them? Every hour matters in terms of revenue, that kind of thing.

IUS: What lies ahead when it comes to aircraft autonomy?
SINGH: Demonstrator aircraft aside, there’s hard work to be done because the aviation industry is a conservative one with good reason. Our focus first went from advancing the art of the possible, to the art of the practical. How do we avoid building a new bespoke system for each new aircraft? And ensure there’s a common autonomy core able to accommodate a wide-range of use cases, with fixed set of variations—so we don’t need to start from scratch every single time?
Now the third mode that we’re in is commercialization and reliability mode. It’s not just possible, and practical, but how can we scale these things and prove their reliability. That’s the evolution of what we’re doing here: It’s no longer just a proof of concept, but a tool we can incorporate into a wide range of tasks.