Discover how Nathan Michael, CTO of Shield AI and former Associate Research Professor at Carnegie Mellon University’s Robotics Institute, is leading the charge in developing resilient intelligent systems capable of autonomous decision-making and navigation in challenging environments, independent of GPS or continuous communications. Learn the details of the Hivemind AI & Autonomy platform that is shaping the battlefield of the future.
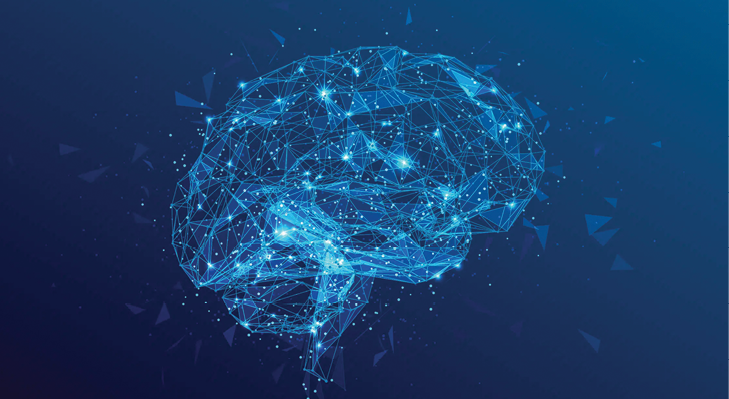
Nathan Michael is Shield AI’s Chief Technology Officer and a former Associate Research Professor in the Robotics Institute of Carnegie Mellon University (CMU). At CMU, Professor Michael was the Director of the Resilient Intelligent Systems Lab, a research lab dedicated to improving the performance and reliability of artificially intelligent and autonomous systems that operate in challenging, real-world and GPS-denied environments. Michael has authored more than 150 publications on control, perception, and cognition for artificially intelligent single and multi-robot systems, for which he has been nominee or recipient of nine best paper awards (ICRA, RSS, DARS, CASE, SSRR).
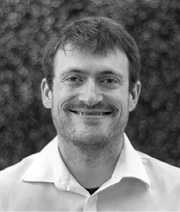
IUS: Could you give a bit of background on your work and what technical specializations you bring to the fold at Shield AI?
NATHAN MICHAEL: My background is in developing resilient intelligent systems. And really what my focus is within the context of Shield AI is bringing that level of intelligence both for single and multi-agent [autonomous] teams to the field, to operational environments and conditions. I oversee the part of the organization that builds out those AI and autonomy capabilities that we call Hivemind.
My focus is on creating that technology, and then enabling the application of that technology to different platforms to meet different customer needs. What we’ve really focused on is creating a technology that allows us to apply sophisticated AI and autonomy capabilities to a variety of different classes of platforms, a variety of different mission sets and scenarios. And to be able to do that with a great deal of flexibility to meet the needs of customers’ particular mission sets, and to have portability in terms of how to apply these AI and autonomy capabilities, even though they’re [applied to] potentially very different classes and platforms.
So, we’ve deployed our AI and autonomy on quadcopters and smaller systems, we’ve deployed on our Group Three system, the VBAT, and Group Five uncrewed systems as well. And we’ve recently started integrating on the Firejet from Kratos, we’re currently working toward integrating onto the Valkyrie, also from Kratos, as well as deploying it on the VISTA and other types of Group Five systems, either crewed or uncrewed.
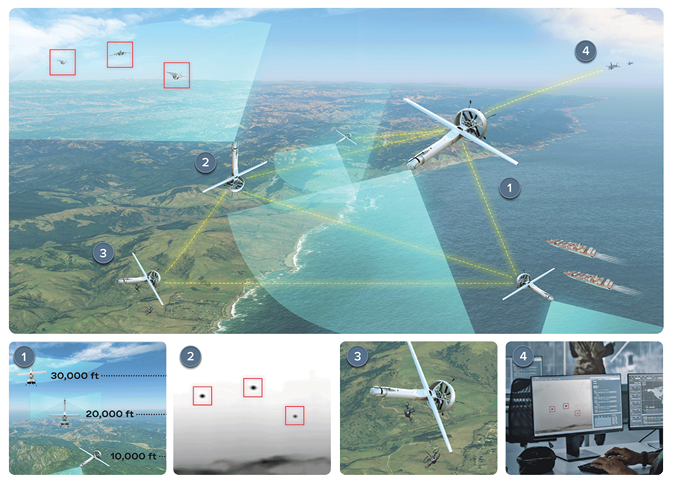
IUS: Can you go into some detail about how the platform is operating in GNSS-denied environments? Could you comment about that, and then more broadly about the operational requirements in a completely denied or jammed environment?
NATHAN MICHAEL: We develop a state estimation framework that allows us to fuse a variety of different sensor observations to yield a consistent state estimate. We are using visual information, the EO/IR thermal [Electro-Optical and Infrared sensors], depending on the platforms that we’re leveraging, and if they’re so equipped, we are using inertial information.
If you go back to the quadcopters, those were very size, weight and power constrained systems that have many different types of sensors, onboard depth cameras, as an example, and so we were taking that information and fusing it together in real time to enable the system to estimate its state in completely denied conditions. This would be GNSS denied as well as communications [denied]. And those types of capabilities are what we’re integrating on board the VBAT at present. We are in the motion of doing that at this time, to be able to support denied operations in the context of comms and GNSS.
We are using INS [inertial navigation] systems, leveraging those capabilities as well.
The state estimation framework…is fusing all that information together to yield a consistent model of the environment. Within the quadcopter case, we also had to create very high precision models of the world around the platform. So, as it navigated in these confined, unknown environments that were denied conditions, the system had to both perceive the world around it, as well as estimate its state within that world and navigate through that world. And often those environments were completely unknown, and not somewhere that had previously been visited. A recent example is the [Shield AI] Nova platforms were used within the tunnels in Gaza, to be able to enter into, clear and explore those environments.
Within the context of Shield AI, we really developed this kind of resilient intelligence concept and deploy that when these systems are operating in highly confined, challenging domains where it’s GNSS denied, comms denied, never been visited by at least the individuals leveraging the systems at that point [in time]. Those systems are onboard, perceiving the world by onboard sensors, estimating its state within that world while also creating the map or the perceptual model of that world. And then, [the system is] navigating through, as it explores and executes against its mission—which is typically to explore the environment in order to find particular items or objects of interest, or just to create a model of the environment and explore it as thoroughly as possible.
IUS: In terms of the VBAT platform, are those capabilities you’ve described in place there?
NATHAN MICHAEL: We’ve taken many of those capabilities and started moving them on to the VBAT, to enable denied operations in environments where there is active jamming. We are in the process of doing that right now. Another area of capability that we’ve developed historically for the smaller systems is the ability for teams of platforms to work together in a coordinated manner where they don’t depend on the existence of any particular platform or any centralized point of contact, because those environments do not necessarily allow for that.
This is this idea of centralized to decentralized coordination and control of multi-agent systems. And we’re now taking that to the VBATs as well. And in that context, we [have already] started demonstrating teaming capabilities with the VBATs, in a prototype context. Now we’re in the process of commercializing the VBAT Teams, and really [making it] robust to the extent that we can start to field it.
IUS: Can you go into more detail about what exactly Hivemind is, and how Hivemind operates from a platform perspective?
NATHAN MICHAEL: Hivemind is how we describe the set of AI and autonomy capabilities that we’re deploying on our platforms. There are four key pillars to it. I use the acronyms of HMC through HMF, so HM [Hivemind] C-D-E-F. Hivemind Commander, which enables the operator to interact and engage with the system…this requires a high degree of consideration to manage cognitive burden as it applies to the operator, particularly when they’re working with many different platforms, where those platforms may or may not be able to communicate at any particular time. And as they’re coordinating together under these challenging conditions.
There’s Hivemind Design, which is the design framework that we leverage to expedite the development of these AI and autonomy capabilities. This is a framework that includes the ability to design scenarios, configure those scenarios, test and evaluate them in simulation with software-in-the-loop or hardware-in-the-loop. [Then] take that through automated analysis pipelines to really understand the performance. And then close the loop with respect to that performance, based on different algorithmic implementations, to improve the actual performance of the AI and autonomy capabilities in a feedback manner.
There’s an Edge [Hivemind E.], which is that edge level intelligence that runs on the platforms themselves. And this is where you’re going to have everything you need to deliver a resilient intelligent system. From a perception, cognition and action perspective, a system that can perceive the world around it, understand that world, think within the context of that world given the mission set across one or multiple platforms, and then ultimately engage with that world and maneuver and act within that world. And so that’s all running on board the platform, and therefore able to operate with or without external communications or external information, by just leveraging the onboard sensors, actuators and communications.
The final part is…the foundations. So that’s HMF, Hivemind Foundations. And that’s this foundational framework that allows us to create these highly scalable, composable AI and autonomy architectures, and to be able to test and evaluate them very precisely. And really drive toward performance on what are size, weight and power constrained systems, depending on the platform.
So that’s what Hivemind is, when you talk about the AI pilot, this is the term that often is used for Hivemind Edge, that edge level intelligence. And if you talk about the AI factory, this is the term that’s often used to describe Hivemind Design. And it’s often phrased…[as] the AI pilot in the AI factory, but this is what I refer to as Hivemind Edge, that edge level intelligence. And Hivemind Design, that ability to design and create a highly performant, edge level intelligence. So that is what Hivemind is.
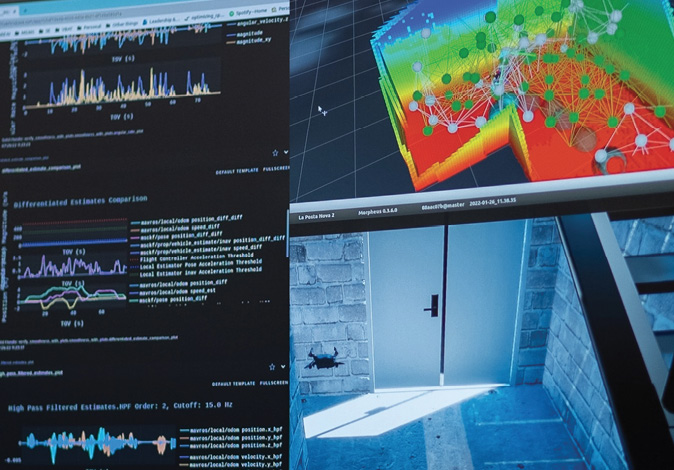
IUS: So, to be clear, when you are talking about Hivemind Edge and perception, cognition and taking action, that is without any datalink at all?
NATHAN MICHAEL: The way to interpret it is communications, GNSS information, operator interaction and engagement—if that information [or] that access is available, it will be used, but it’s not necessary. So as an example, to go back to that underground tunnel within Gaza, they’re dropping [Hivemind-enabled Nova] in, there’s obviously underground no GNSS link, pretty quickly you’re going to lose your comms link. And that system onboard is able to perceive the world around it, think completely onboard without any external interaction or engagement, make decisions based on where to go, what to do, how to operate, keep track of its own vehicle health, and then ultimately make decisions on how to progress against the mission. And then once the battery level and the endurance are at a certain point, it will take certain actions to pace based on what the inputs are from the operator before we even went on the mission. But probably it will come back to where it started.
Resilient intelligence is the ability for a system to think for itself and operate without requiring external inputs to introspect, adapt and evolve. And to be able to do so both across one or many agents in a system. So, when I described that single agent in the tunnel operating underground without any human interaction, it could be one or many agents. Within an academic context, I used to deploy systems that were three of them operating all the way up to 50. The key point here is creating the ability for scalable, resilient intelligence that operates at the edge and…creating a system that can truly think for itself to achieve the [human-defined] mission.
IUS: Can you talk a little bit about interoperability and integration? And how Hivemind and AI pilot-enabled systems become deployed? What about some of those integration challenges in interoperability with other systems?
NATHAN MICHAEL: Let me just walk through the lifecycle. If I want to apply Hivemind, I have those four parts that I described. Of course, the edge level intelligence, or you’re referring to it as AI pilot, but they’re synonymous, that edge level intelligence is built on the foundations. And that edge level intelligence, in general, is based off common reference implementations. But it’s this highly modular, granular system that allows us to compose and modify, while still maintaining a high degree of quality control.
When I step up to a new platform, to integrate that AI pilot, I really just need to bridge between the sensor data being inputted into that system, into the appropriate pipelines within that AI and autonomy architecture. And similarly, I need to integrate with the actuation system and the I/O system on there to just close that feedback [loop].
We specifically designed our Hivemind Foundations to be highly interoperable and really extensible. That means for us to be able to connect into native protocols or native ways that different platforms communicate, it’s actually extremely straightforward. For us to be able to hop on to a new platform, consume new sensor data, interact and close that loop is actually really straightforward.
We do have to go through that development effort if we haven’t already done it. But otherwise, in general, all the different blocks or components or modules within that AI and autonomy architecture that’s running [Hivemind] Edge remains largely unchanged.
We have a modular state estimation framework, as an example, a modular mapping framework. Also, a modular cognition framework, modular executive management framework…And a modular motion manager, effects manager framework that allows us when we enter into the system to be highly extensible, highly configurable, and just surgically add in the necessary plugins to interface within the context of that platform.
The second point is, we have this Hivemind Design framework, which I also called the AI factory before, that actually is co-architected with the Hivemind Edge capabilities, to allow us to really test and rigorously evaluate the performance of this new intelligence onto this new platform. We have plugin-based simulators, plugin-based analyzers.
Even though we’re walking up to a new platform with new sensors and new capabilities, we’re very readily able to configure and extend that framework, allow[ing] us to rigorously test across a variety of different scenarios. Then ultimately, we’re able to establish a data driven approach to analyzing the performance of this edge level intelligence. And we’re able to progress from simulation-based software-in-the-loop to hardware-in-the-loop to eventually then iteration on the platform. And this is all very straightforward to do.
We’ve gone through this motion now quite a few times. The first time we applied it to Nova One, that probably took us about 36 months. By the time we went to Nova Two it was more like 18 to 24 [months]. By the time we get to the VBAT, it took us about 12 months. And with the [Kratos] Firejet, it took us about three to four months. And with the [Kratos] Valkyrie it’s going to be much faster.
So, with each new platform, we’re getting our framework dialed in, getting our approach dialed in. And we’re able to turn those cycles much, much more rapidly. So ultimately, then we’re deploying the capabilities on the real platform. And increasing the sophistication of that evaluation, getting the feedback from that, [then] running it through that Hivemind Design, the [AI] factory, to evaluate that performance and engage in quality control, and then iterate rapidly to improve that performance.
That’s the end-to-end motion to go from, ’here’s a new platform, make it intelligent’, one or more of them, to actually deploy that in the real world. And as I said, at this point, we’ve gotten that motion down to as quick as about three to six months. And I think if we had to work with a new platform, it would probably be around that order of three to four months at this point. So that’s sort of that motion.
IUS: This compression of the implementation timeline that you’ve described, you’ve compressed it from 36 to three months. Does this have to do with the kind of machine learning and reinforcement learning techniques that you’re applying?
NATHAN MICHAEL: Quality in the nature of the infrastructure and just getting everything dialed in. You have all the software dialed in, all the implementations you need to deploy resilient intelligence, that’s the AI pilot. You have all the capabilities and infrastructure you need to develop and deliver that resilient intelligence, that’s the AI factory. And just getting that so dialed in to the point where now, if you need to extend and configure that system to be able to deploy intelligence, you can do it very, very quickly. It is at that point where it is sufficiently extensible, sufficiently configurable and really interoperable.
So we are able to drive fast iteration cycles by leveraging AI/ML for improvement, particularly if you think of it as testing and verification in a simulation, or [within] a software/ hardware context. That’s all part of that story. But yes, a combination of really getting the edge level intelligence, so the AI pilot, the frameworks and the infrastructure, the AI factory, dialed in, and leveraging innovative techniques that really make it possible to go fast in terms of development and the deployment.
IUS: Can you describe a bit more about interoperability with other systems?
NATHAN MICHAEL: I think there’s a number of ways to think about interoperability. There is the ability to support new sensors, new platforms. The ability to support new types of protocols, which is why we designed that foundational framework to be highly extensible. We can add new protocols very readily to it, and nothing else has to change for everything else we did. And that means if you have to talk to another middleware, another provider, another platform, we fully expect that, and we can support that readily. And then there’s the interoperability with other broader ecosystems.
And we’ve really designed with that in mind because that is really important for the customer. And so, we have, again, extensibility, and the ability to adapt to support new protocols, new ecosystem integrations. And then finally, there’s interoperability from an AI and autonomy perspective, in terms of different types of platforms working with [other] types of platforms. And we have always architected our AI and autonomy to support heterogeneity. That’s baked into the system overall.
IUS: There’s a real demand and requirement now for modularity and open systems, open architecture. The DOD wants modularity. And rather than having very large incumbent primes own the entire engineering value chain, there’s this willingness and requirement now, in fact, due to the geopolitical environment we live in, to diversify.
NATHAN MICHAEL: For sure. And we’ve designed for that from day one. That has always been part of the fabric. And that’s why we created that foundational framework that I described, because it makes it extremely accessible to permute and modify, and swap one part out with another part. And it allows for the design and development of a highly MOSA-compliant [modular open systems approach] system.
And with the factory component to it, what it allows for is the end user, the non-subject matter expert to…engage and interact with [the platform]. One of the big capabilities or value deliveries that we’re working on right now is to take what would require a high degree of sophistication, and engineering proficiency and expertise, and make that accessible to non-subject matter experts, and make it possible to really think in terms of introducing new capabilities, whether it’s the developer that wants to add in functionality and swap out what we’re developing, or it’s the operator that wants to change from day to daythe behaviors or the tasks that the team are executing. And making it possible to deploy that level of flexibility while maintaining a high degree of quality control, and assurance and trustworthiness of the actual delivered capability itself. This idea of creating highly extensible, highly modular, highly configurable, readily deployable and portable capabilities is essential to what we were going after from day one in terms of the architecture and what we formulate.
IUS: In the past, people have thought more about swarms as kind of relative positioning and this kind of network effect, whereas your approach is more sophisticated, more complex. Can you talk a little bit about the trend toward swarming, what you envision as the capability of swarming, and how it may drive change on future engagement on the battlefield?
NATHAN MICHAEL: There are many different ways to talk about swarming. One could be thinking of it as…large numbers of highly intelligent systems that are capable of working with each other. And you could talk about teams that go anywhere from many to one. And that is to say, under those worst-case conditions, you’ll end up having one intelligence system that’s having to operate, and then as they can communicate with each other, if it’s possible, then they can start to coordinate. But if it’s not possible, then they’re unable to.
So, creating these resilient teams that are able to operate across a variety of different mission sets and domains. That’s what I mean when I talk about teaming and the scale of those teams, which moves toward swarming. What we’re really trying to do is create these highly sophisticated and capable intelligent teams that are working together proficiently and adapting and resilient to changes and conditions.
IUS: Thinking about the fact you’ve got this resilient teaming capability, where one platform is not related to another, that they are intelligent beings that can deploy and reorganize and organize on the fly, how do you see that changing the nature of warfare? Generally, from your perspective, what is the future vision? What does it look like, and what’s the impact?
NATHAN MICHAEL: It looks like from a true fielding perspective, a much smaller commitment of personnel to effect and achieve a much greater mission outcome. I think at the essence of that, or at the fundamental level, that’s a statement that we are putting fewer people in harm’s way…minimizing risks to personnel while maximizing efficacy, efficiency, scale. And I think when you have this level of intelligence, the efficiency and efficacy aspect of it also increases. So, when you think about the first point, which is fewer people in harm’s way, fewer people at risk. The second point, I think doubles down on that, because it says, now you have more intelligent systems, enabling the processing and the assessment of conditions to make even better decisions, and to enable the operator and those reduced numbers of persons to make even better decisions at scale. And so, this means that we are achieving greater outcomes with less consequential costs.
IUS: And it also means it potentially changes tactical considerations and strategic considerations for the DOD. It’s extraordinarily transformative. Do you get a sense of that? Do you feel the weight of that work?
NATHAN MICHAEL: I think at this stage, the reality is, we have a team of engineers that are extremely capable. And, you know, they have many options. But the reason why they choose to work on this problem set is because to the person, they understand the significance of what we’re striving to achieve. I think it was probably a month ago, I met with the engineering team working on this and basically said [to them], the finite amount of time that we have, the importance of what the mission is, and the level of commitment that we must maintain and hold. And the standards to which we must hold ourselves as we pursue that commitment. That is very reinforcing for our team because it really helps bring focus and clarity to the value of what the team is doing. But also, it creates a reasonable amount of pressure in terms of the pace at which we need to keep moving forward to make sure that we rise to the occasion and meet the call.