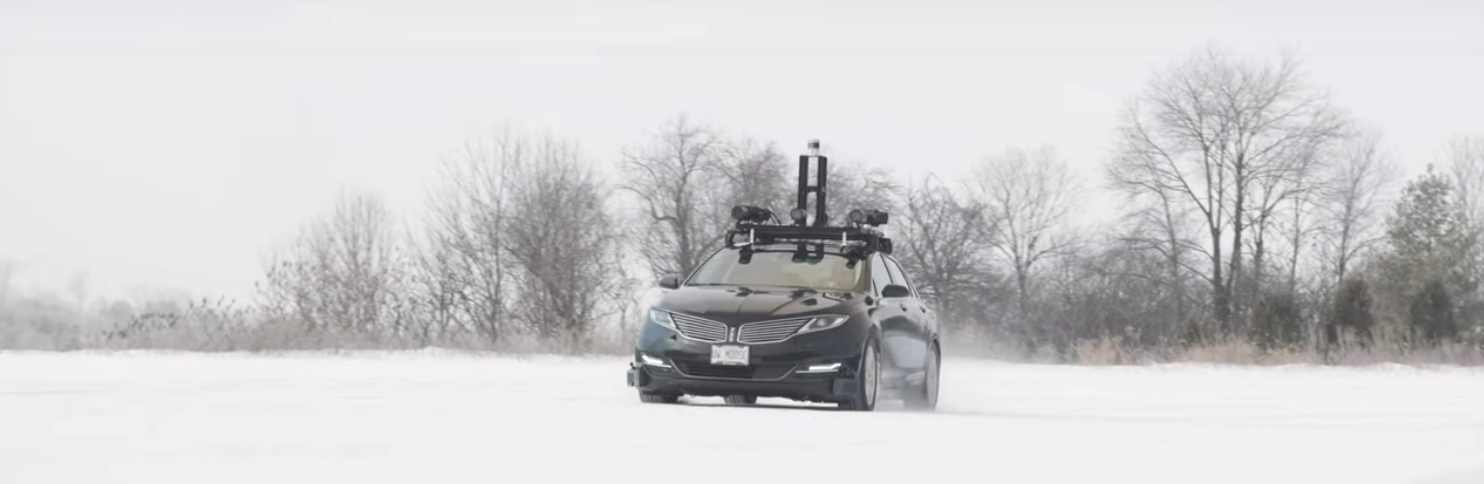
Autonomoose. Photo courtesy University of Toronto and University of Waterloo.
A Lincoln MKZ hybrid carrying a full sensor suite roves the snowy roads of Ontario, recording bad-weather data to empower driverless vehicles that will eventually brave these conditions. Driving algorithms generated by data collected in good weather can become confused, and miscalculate badly, because of different visual conditions in snowy weather with extensive white backgrounds.
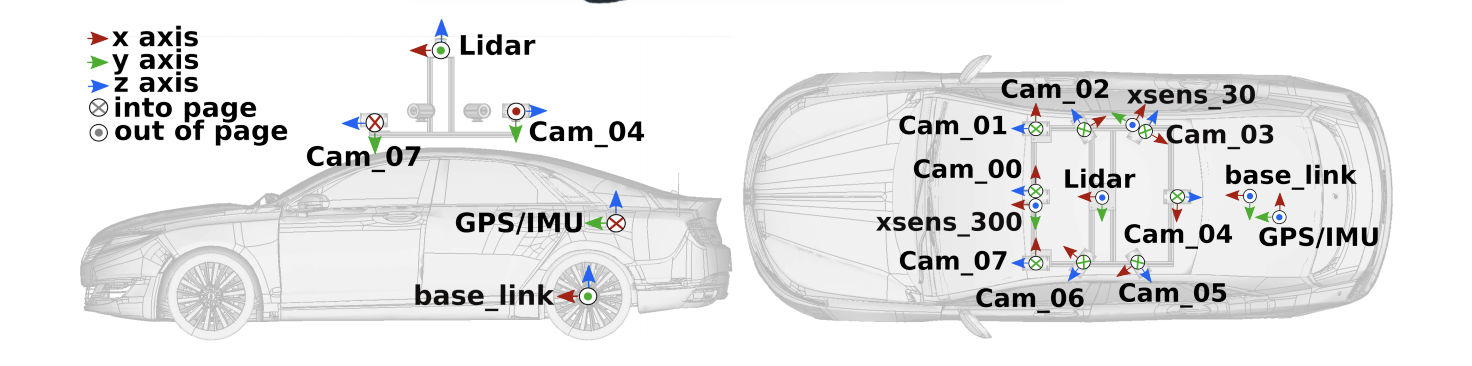
Autonomoose sensor suite. Courtesy U. Toronto and U. Waterloo Canadian Adverse Driving Conditions project.
The Autonomoose’s suite of synchronized sensors includes eight cameras (Ximea MQ013CG-E2), a LiDAR scanner (Velodyne VLP-32C), a GPS/inertial system (Novatel OEM638, incorporating a Sensonor STIM300 MEMS IMU), two additional IMUs (Xsens MTi-300- and 30-AHRS) as part of the advanced driver assistance system (ADAS). The suite records and timestamps 10 images per second.
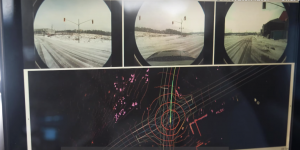
Autonomoose image collection. Courtesy U. Toronto and U. Waterloo Canadian Adverse Driving Conditions project.
The LiDAR is time-synchronized with the GPS PPS signal and NMEA messages. Each LiDAR point cloud contains a full 360° sweep of the LiDAR beams, starting from the 180° cut angle directly behind the car and rotating clockwise. The Autonomoose computer receives the GPS PPS signals and NMEA messages. A Novatel Inertial Position Velocity Attitude-Extended (INSPVAX) message contains the most recent position, velocity and orientation as well as standard deviations.
Steven Waslander, an associate professor at the University of Toronto Institute for Aerospace Studies in the Faculty of Applied Science & Engineering, and Krzysztof Czarnecki, a professor at the University of Waterloo, lead the team compiling the Canadian Adverse Driving Conditions dataset. A collaboration with Scale AI helps them categorize the data.
“Data is a critical bottleneck in current machine-learning research,” said Alexandr Wang, Scale AI CEO. “Without reliable, high-quality data that captures the reality of driving in winter, it simply won’t be possible to build self-driving systems that work safely in these environments.”
The project has placed the data, documentation and support tools in GitHub. An open-access scientific article on arXiv explains the data collection process. The dataset contains 7,000 frames collected through a variety of winter driving conditions. Lidar frame annotations that represent ground truth for 3D object detection and tracking have been provided by Scale AI.
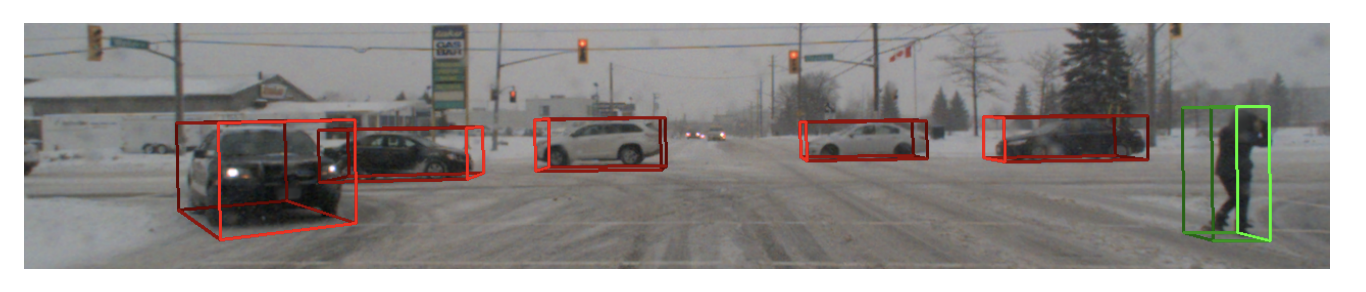
Each cuboid from the 3D annotation file is projected onto the
image of the front camera. Courtesy U. Toronto and U. Waterloo.
“Bad weather is a condition that is going to happen,” said Waslander. “We don’t want Canada to be 10 or 15 years behind simply because conditions can be a bit tougher up here.”
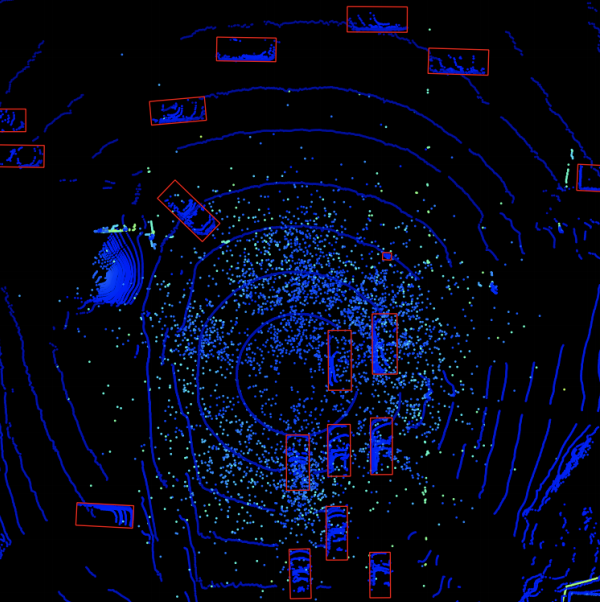
Each cuboid from the 3D annotation file is displayed within
the lidar frame from a top down perspective. Courtesy U. Toronto and U. Waterloo Canadian Adverse Driving Conditions project.